A Handwritten Digit Recognition Method Based on Improved Spiking Neural Network
A spiking neural network, digital recognition technology, applied in the field of image recognition, can solve the problems of increasing the recognition process time, time-consuming, complex calculation, etc., to achieve the effect of improving the recognition accuracy rate and training speed
- Summary
- Abstract
- Description
- Claims
- Application Information
AI Technical Summary
Problems solved by technology
Method used
Image
Examples
Embodiment Construction
[0060] Such as figure 1 As shown, a handwritten digit recognition method based on an improved spiking neural network, including:
[0061] Step 1. Input and encode the MNIST dataset, which is divided into two steps:
[0062] (1.1) Import the training samples, test samples and label data of the MNIST data set;
[0063] (1.2) A pulse train with a Poisson distribution is input to the network, and the firing rate of the pulse train is proportional to the pixel intensity of the MNIST dataset image.
[0064] Step 2. Establish a spiking neural network model and determine the connection mode between neurons, which is divided into two steps:
[0065] (2.1) Determine the spiking neural network structure for handwritten digit recognition, such as figure 2 As shown, the first layer is the input layer, which contains 28×28 neurons (each neuron represents an image pixel), and the second layer is the processing layer, which contains 400 excitatory neurons and the same number of inhibitory...
PUM
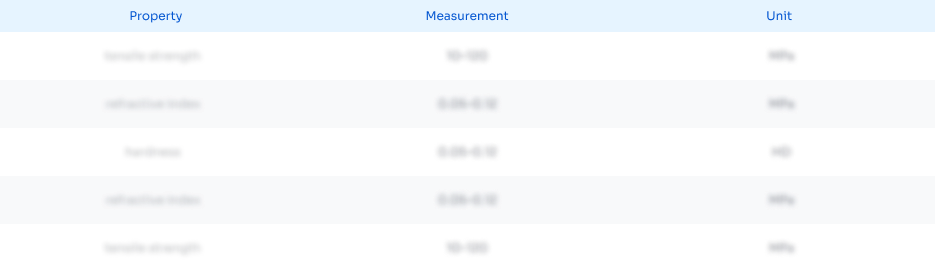
Abstract
Description
Claims
Application Information
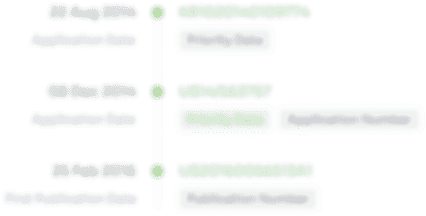
- R&D Engineer
- R&D Manager
- IP Professional
- Industry Leading Data Capabilities
- Powerful AI technology
- Patent DNA Extraction
Browse by: Latest US Patents, China's latest patents, Technical Efficacy Thesaurus, Application Domain, Technology Topic, Popular Technical Reports.
© 2024 PatSnap. All rights reserved.Legal|Privacy policy|Modern Slavery Act Transparency Statement|Sitemap|About US| Contact US: help@patsnap.com