Edge server joint task unloading and convolutional neural network layer scheduling method
A convolutional neural network, edge server technology, applied in data exchange networks, digital transmission systems, electrical components, etc., can solve the problem of limited network transmission performance, less consideration of delay optimization, and algorithms that are difficult to optimize network performance, etc. problem, to achieve the effect of network performance optimization and service quality improvement
- Summary
- Abstract
- Description
- Claims
- Application Information
AI Technical Summary
Problems solved by technology
Method used
Image
Examples
Embodiment Construction
[0034] The preferred embodiments of the present invention will be described in detail below with reference to the accompanying drawings.
[0035] An edge server joint task unloading and convolutional neural network (CNN) layer scheduling method described in the present invention assumes that there are certain tasks to be executed in the user equipment, and the edge server deploying CNN has a certain task processing capability to meet the task unloading constraints And CNN layer scheduling constraints as the premise, the user will choose the appropriate edge server to offload the task, and the edge server can flexibly change the number of calling layers of the multi-layer CNN deployed on it, and balance the processing delay of the tasks offloaded to the edge server and transmission delay to minimize the total task delay. The modeling takes the total task delay as the optimization goal, optimizes and determines the edge server task offloading and CNN layer scheduling strategy, and...
PUM
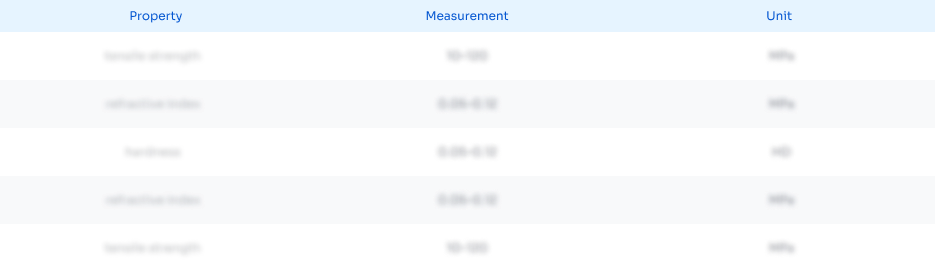
Abstract
Description
Claims
Application Information
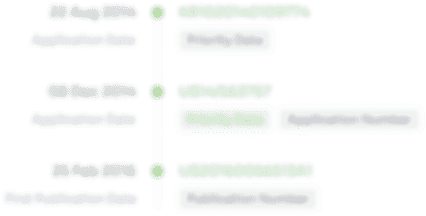
- R&D Engineer
- R&D Manager
- IP Professional
- Industry Leading Data Capabilities
- Powerful AI technology
- Patent DNA Extraction
Browse by: Latest US Patents, China's latest patents, Technical Efficacy Thesaurus, Application Domain, Technology Topic, Popular Technical Reports.
© 2024 PatSnap. All rights reserved.Legal|Privacy policy|Modern Slavery Act Transparency Statement|Sitemap|About US| Contact US: help@patsnap.com