Deep learning-based multiview face three-dimensional model reconstruction method
A three-dimensional model and deep learning technology, applied in the field of computer vision, can solve the problems of slow processing speed, unsatisfactory effect of restoring the detailed features of the face, etc., and achieve the effect of fast speed
- Summary
- Abstract
- Description
- Claims
- Application Information
AI Technical Summary
Problems solved by technology
Method used
Image
Examples
Embodiment
[0051] This embodiment provides a multi-view face 3D model reconstruction method based on deep learning, see figure 1 , including:
[0052] (1) Use the 3DMM face database of Basel Face Model to randomly generate 2300 face models with neutral expressions, of which 1800 models are used as training sets and 500 models are used as test sets. There are 9 types of virtual lighting and 5 camera perspectives (rotation matrices). Establish a virtual viewpoint projection to obtain a corresponding two-dimensional picture. From the 2300 face models, 2300×9×5 pictures of different faces under different lighting and different viewing angles can be obtained. Before entering the network, data enhancement processing is performed on the data, including random adjustment of contrast within a certain range, etc., so that the training samples are more abundant and the results are more robust.
[0053] (2) Select the camera internal and external parameters of the front view angle (0° angle of vi...
PUM
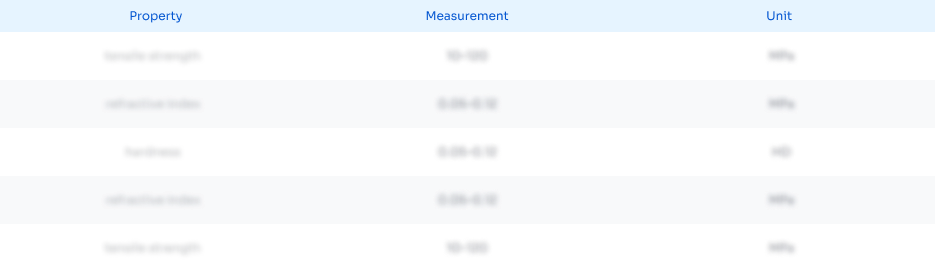
Abstract
Description
Claims
Application Information
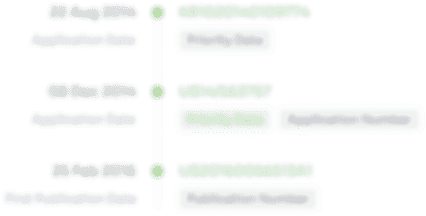
- R&D Engineer
- R&D Manager
- IP Professional
- Industry Leading Data Capabilities
- Powerful AI technology
- Patent DNA Extraction
Browse by: Latest US Patents, China's latest patents, Technical Efficacy Thesaurus, Application Domain, Technology Topic, Popular Technical Reports.
© 2024 PatSnap. All rights reserved.Legal|Privacy policy|Modern Slavery Act Transparency Statement|Sitemap|About US| Contact US: help@patsnap.com