Fault Diagnosis Method of Bearings Based on Average Multiple Granularity Decision Rough Sets and nnbc
A fault diagnosis, multi-granularity technology, applied in the testing of machine/structural components, testing of mechanical components, instruments, etc., can solve problems such as inappropriate selection of constraints, and achieve improved clustering effect, accurate fault type and fault degree , the effect of reducing computational complexity
- Summary
- Abstract
- Description
- Claims
- Application Information
AI Technical Summary
Problems solved by technology
Method used
Image
Examples
specific Embodiment approach 1
[0033] Specific implementation mode one: combine figure 1 Illustrate this embodiment, based on average multi-granularity decision-making rough set and NNBC bearing fault diagnosis method, it is characterized in that the method comprises the following steps:
[0034] Step 1, extracting the fault diagnosis features of rolling bearings in the training samples to construct a fault symptom attribute set;
[0035] Step 2. Using the attribute reduction algorithm based on the average multi-granularity decision-making rough set to reduce the dimensionality of the symptom attribute set in the training sample;
[0036] Step 3: Construct NNBC based on the reduced training samples, and use the NNBC-based pattern recognition algorithm to judge the rolling bearing status of the samples to be diagnosed.
specific Embodiment approach 2
[0037] Specific embodiment two: the difference between this embodiment and specific embodiment one is: the attribute reduction algorithm based on the average multi-granularity decision-making rough set in the step two, combined with figure 2 Describe this implementation mode:
[0038] Step 21. According to the risk price λ p,q (p,q=1,2,3), calculate the threshold α; where
[0039] Step 22. Calculate the classification quality S of the symptom attribute set C with respect to the decision attribute D C (D);
[0040] Step two and three, in turn for each symptom attribute c i ,i=1,2,...,n perform the following operations;
[0041] Step 24: Calculate symptom attribute c i In the symptom attribute set C, the importance Sig(c i ,C,D);
[0042] Step 25, if the symptom attribute c i The importance of Sig(c i ,C,D)=0, then the symptom attribute c i is redundant, otherwise essential;
[0043] Step 26. Repeat steps 24 and 25 for other symptom attributes until the last sympto...
specific Embodiment approach 3
[0045] Embodiment 3: The difference between this embodiment and Embodiment 1 or 2 is: the classification quality S of the symptom attribute set C with respect to the decision attribute D in the step 22 C (D) is defined as follows:
[0046] For the information system S=(U,A=C∪D,V,f), U is the instance set, A is the attribute set, C is the symptom attribute set, D is the decision attribute set, V is the range of A, f is Mapping function, let B={B 1 ,B 2 ,...,B m} are m attribute subsets of symptom attribute set C, then the classification quality of symptom attribute set C with respect to decision attribute D is:
[0047]
[0048] in, is the lower approximate set of the average multi-granularity decision rough set of set X about B, and α is the threshold.
PUM
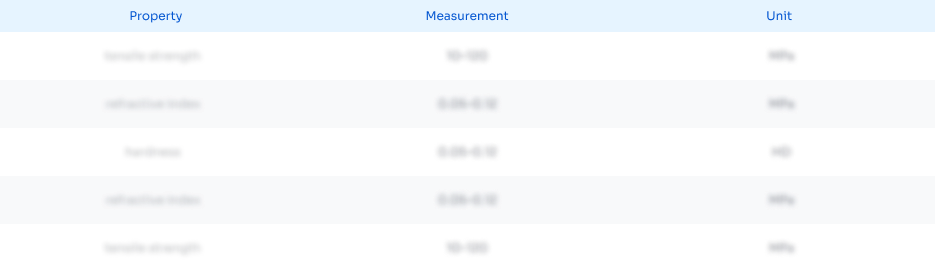
Abstract
Description
Claims
Application Information
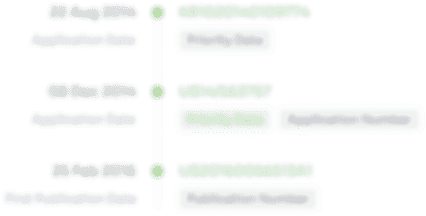
- R&D Engineer
- R&D Manager
- IP Professional
- Industry Leading Data Capabilities
- Powerful AI technology
- Patent DNA Extraction
Browse by: Latest US Patents, China's latest patents, Technical Efficacy Thesaurus, Application Domain, Technology Topic, Popular Technical Reports.
© 2024 PatSnap. All rights reserved.Legal|Privacy policy|Modern Slavery Act Transparency Statement|Sitemap|About US| Contact US: help@patsnap.com