A defect detection method for granular products based on deep learning
A technology for product defects and detection methods, which is applied in the application of machine vision and deep learning technology in product inspection, especially in the detection of pharmaceutical products. In the field of particle product defect detection based on deep learning, it can solve the problem of high false detection rate and missed detection rate. , high requirements for irradiation accuracy, narrow use range and other problems, to achieve the effect of low false detection rate and missed detection rate, reduce production cost and improve production efficiency
- Summary
- Abstract
- Description
- Claims
- Application Information
AI Technical Summary
Problems solved by technology
Method used
Image
Examples
Embodiment Construction
[0035] The present invention will be described in detail below with reference to the accompanying drawings and the embodiments thereof, but the protection scope of the present invention is not limited to the scope described in the embodiments. Those skilled in the art should understand that although the following description takes the detection of tablets as an example, the method of the present invention is not limited to the detection of drugs, but can also be applied to products with similar packaging and structures, especially granular products. For example, candy, chocolate, etc.
[0036] Step 1, target image capture:
[0037] Because the defect scale of the tablet object is relatively small, a high-resolution industrial camera is selected for shooting in this embodiment. The camera is placed directly above the conveyor belt, and the medicine is placed in the drug board during inspection, and the side with the transparent blister is facing up the conveyor belt. With the ...
PUM
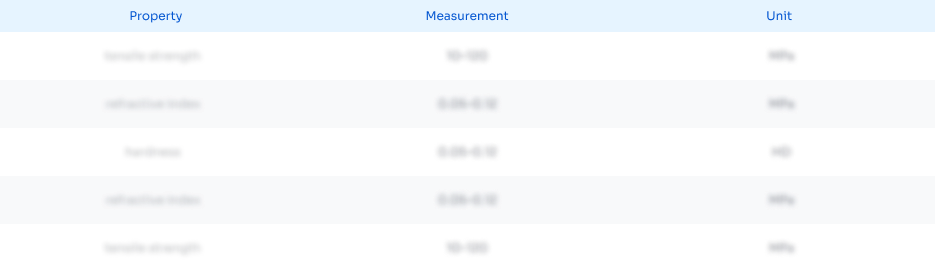
Abstract
Description
Claims
Application Information
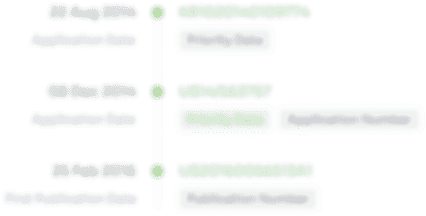
- R&D Engineer
- R&D Manager
- IP Professional
- Industry Leading Data Capabilities
- Powerful AI technology
- Patent DNA Extraction
Browse by: Latest US Patents, China's latest patents, Technical Efficacy Thesaurus, Application Domain, Technology Topic, Popular Technical Reports.
© 2024 PatSnap. All rights reserved.Legal|Privacy policy|Modern Slavery Act Transparency Statement|Sitemap|About US| Contact US: help@patsnap.com