Neural network-based manually annotated white blood cell error calibration method and device
A neural network and manual labeling technology, applied in the field of computer vision, can solve the problems of high misdiagnosis rate, small number of cell classifications, cumbersome procedures, etc., and achieve the effect of increasing the accuracy rate and reducing the time for manual judgment.
- Summary
- Abstract
- Description
- Claims
- Application Information
AI Technical Summary
Problems solved by technology
Method used
Image
Examples
Embodiment 1
[0032] This embodiment provides a neural network-based error calibration method for manual labeling of white blood cells, see Figure 6 , including:
[0033] S1: Map the original RGB image into the HSV space and separate the S channel.
[0034] Microscopic images of peripheral blood smears include white blood cells, red blood cells, and platelets, among others. After staining, the white blood cell nuclei and platelets are purple, the white blood cell plasma is light purple, and the red blood cells are light pink. Use the DP27 camera to take pictures of blood cells and send them to the computer to obtain the original RGB images.
[0035] First try to separate the RGB channels, and found that under these three channels, various types of cells in the image cannot be effectively distinguished. Map the RGB image to the HSV space, and separate the three channels of H, S, and V. It is found that in the S channel, the discrimination of various blood cells is the highest.
[0036] ...
Embodiment 2
[0070] see Figure 7 , this embodiment provides a neural network-based error calibration device for artificially labeling white blood cells, including:
[0071] An original image acquisition module 201, configured to acquire an original microscopic image of white blood cells;
[0072] This device uses a combination of an Olympus microscope and a DP27 camera to acquire raw images. Under the condition of magnification of 400 times, the image was collected after adding immersion oil, and a tif image with a resolution of 2448×1920 was obtained.
[0073] An image preprocessing module 202, configured to locate the white blood cell part in the blood cell microscopic image;
[0074] Specifically, taking a blood smear taken under a 400X microscope as an example, one picture contains several white blood cells and numerous red blood cells. Firstly, the white blood cells are roughly segmented from the large image using the threshold value, and saved as a picture of a specified size. Th...
PUM
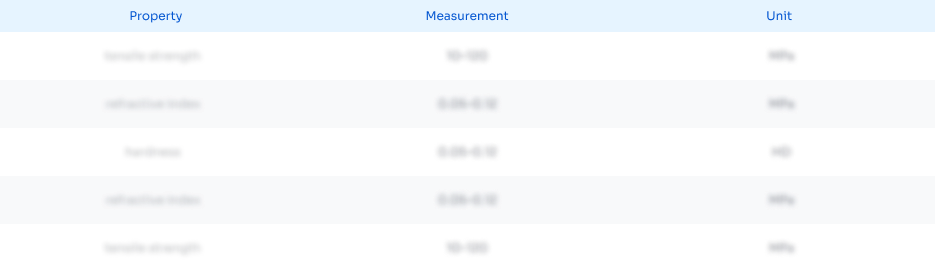
Abstract
Description
Claims
Application Information
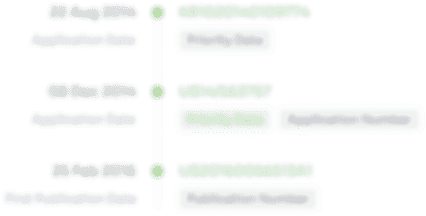
- R&D Engineer
- R&D Manager
- IP Professional
- Industry Leading Data Capabilities
- Powerful AI technology
- Patent DNA Extraction
Browse by: Latest US Patents, China's latest patents, Technical Efficacy Thesaurus, Application Domain, Technology Topic, Popular Technical Reports.
© 2024 PatSnap. All rights reserved.Legal|Privacy policy|Modern Slavery Act Transparency Statement|Sitemap|About US| Contact US: help@patsnap.com