An identification method of catenary key components based on deep learning
A technology of deep learning and recognition methods, applied in computer parts, character and pattern recognition, biological neural network models, etc., can solve problems such as poor generalization ability, low training recognition efficiency, and excessive image exposure, and reduce false detection. rate and missed detection rate, improve the efficiency of training recognition, and reduce the effect of training time
- Summary
- Abstract
- Description
- Claims
- Application Information
AI Technical Summary
Problems solved by technology
Method used
Image
Examples
Embodiment Construction
[0065] In order to make the purpose, technical solution and advantages of the present invention clearer, the present invention will be further elaborated below in conjunction with the accompanying drawings.
[0066] In this example, if Figure 1-8 As shown, a deep learning-based catenary key component identification method includes the following steps:
[0067] (1) Use the catenary suspension state monitoring and detection system (4C) to collect target images to form an original image sample set.
[0068] (2) Image data enhancement: use grayscale transformation and image processing methods of rotation, shearing, mirroring, and noise to process data, expand the data set, form an enhanced image sample set, and combine the enhanced image sample set with the original image sample set Integrate to form a training set, and extract a part of the training set as a verification set and a test set. During the training process, the training set can be expanded at a low cost as the model...
PUM
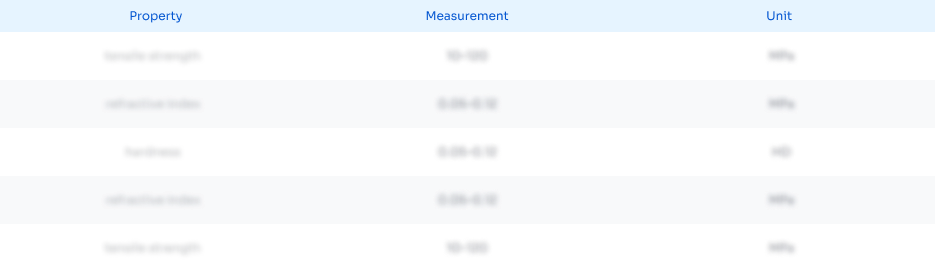
Abstract
Description
Claims
Application Information
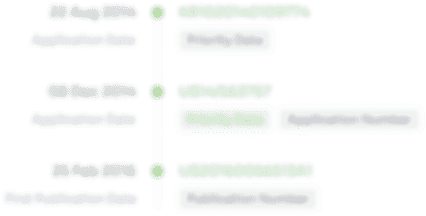
- R&D Engineer
- R&D Manager
- IP Professional
- Industry Leading Data Capabilities
- Powerful AI technology
- Patent DNA Extraction
Browse by: Latest US Patents, China's latest patents, Technical Efficacy Thesaurus, Application Domain, Technology Topic, Popular Technical Reports.
© 2024 PatSnap. All rights reserved.Legal|Privacy policy|Modern Slavery Act Transparency Statement|Sitemap|About US| Contact US: help@patsnap.com