Image retrieval method based on hierarchical convolutional neural network
A convolutional neural network and image retrieval technology, which is applied in the field of deep learning algorithms and image retrieval, can solve the problems of easily ignoring details in retrieval results, lack of data learning process and semantic information cognition, and falling into local optimum.
- Summary
- Abstract
- Description
- Claims
- Application Information
AI Technical Summary
Problems solved by technology
Method used
Image
Examples
Embodiment Construction
[0059] refer to figure 1 , shows a flowchart 100 of the image retrieval method based on the layered convolutional neural network of the present invention, and the specific steps are as follows:
[0060] Step 101, for the input all-sky aurora image database, use the adaptive polarization fence method to determine k local key points of the all-sky aurora image, and obtain the position information of each key point.
[0061] (1a) All-sky aurora image database D={I 1 , I 2 ,...,I N} is the input of layered convolutional neural network, where, I n (n=1,...,N) is the nth image in the above-mentioned all-sky aurora image database, and N is the total number of images in the above-mentioned all-sky aurora image database.
[0062] Preset the parameters of the adaptive polarization fence method: the reference radial interval △ρ is set to 25.6, the reference angular interval △θ is set to π / 4, the parameter v controlling the radial coordinate distribution is set to 0.2, and the control...
PUM
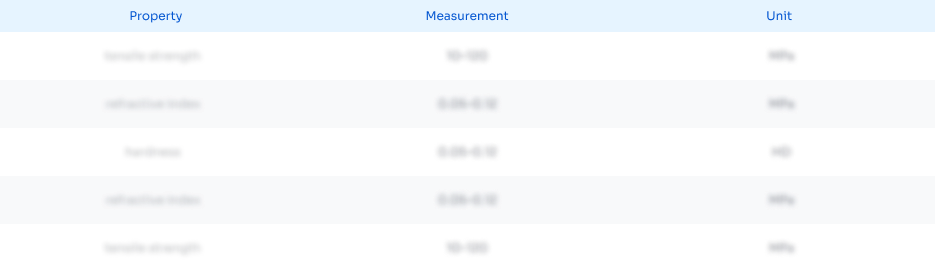
Abstract
Description
Claims
Application Information
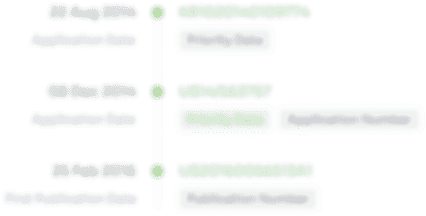
- R&D Engineer
- R&D Manager
- IP Professional
- Industry Leading Data Capabilities
- Powerful AI technology
- Patent DNA Extraction
Browse by: Latest US Patents, China's latest patents, Technical Efficacy Thesaurus, Application Domain, Technology Topic, Popular Technical Reports.
© 2024 PatSnap. All rights reserved.Legal|Privacy policy|Modern Slavery Act Transparency Statement|Sitemap|About US| Contact US: help@patsnap.com