A multi-classification method for heart sounds based on deep convolutional neural network
A neural network and deep convolution technology, applied in stethoscope, medical science, diagnosis, etc., can solve the problems of low resolution performance and low classification accuracy rate, achieve low data length requirements, enhance robustness, and improve classification accuracy rate Effect
- Summary
- Abstract
- Description
- Claims
- Application Information
AI Technical Summary
Problems solved by technology
Method used
Image
Examples
Embodiment 1
[0039] A heart sound multi-classification method based on a deep convolutional neural network, comprising the steps of:
[0040] Step 1: Process the acquired original heart sound data to obtain N-segment heart sound signals;
[0041] Step 2: Input N segments of heart sound signals into the heart sound classification model based on two-dimensional convolutional neural network and one-dimensional convolutional neural network to classify according to frequency domain and time domain features to obtain 2N classification results;
[0042] Step 3: Use the Lasso framework to train the 2N classification results to obtain the corresponding weights, and multiply the weights by the 2N classification results to complete the regression and obtain the final classification results.
Embodiment 2
[0044] Step 1.1: Use an electronic stethoscope with a microphone to collect the heart sound data, and at the same time extract part of the data from the standard data set, and integrate the heart sound data and part of the data to obtain the original heart sound data;
[0045] Step 1.2: Denoising the original heart sound data through a bandpass filter to obtain a cleaned heart sound signal;
[0046] Step 1.3: select several cycles from the multiple heartbeat cycles in the heart sound signal after cleaning to complete the segmentation of the heart sound signal;
[0047] Step 1.4: Move the starting point of the segment randomly left and right, as the final starting point of the heart sound signal segment, and obtain N segment heart sound signals after data augmentation;
[0048] Step 2.1: Perform short-time Fourier transform on the N segments of heart sound signals in chronological order to obtain the spectrogram, and send the spectrogram into the heart sound classification mode...
PUM
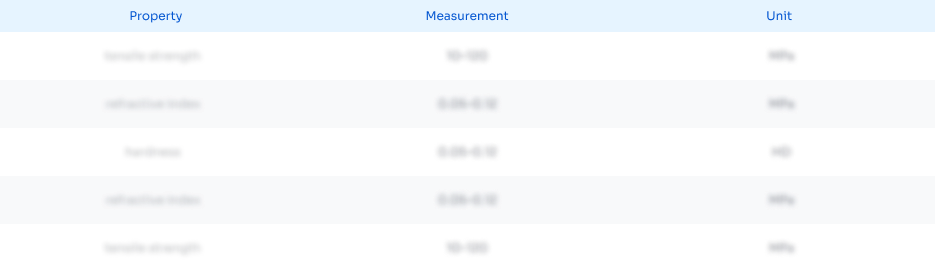
Abstract
Description
Claims
Application Information
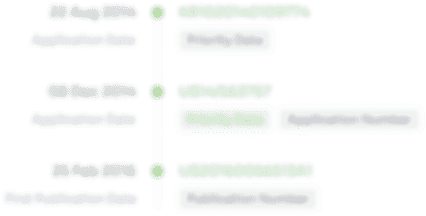
- R&D Engineer
- R&D Manager
- IP Professional
- Industry Leading Data Capabilities
- Powerful AI technology
- Patent DNA Extraction
Browse by: Latest US Patents, China's latest patents, Technical Efficacy Thesaurus, Application Domain, Technology Topic, Popular Technical Reports.
© 2024 PatSnap. All rights reserved.Legal|Privacy policy|Modern Slavery Act Transparency Statement|Sitemap|About US| Contact US: help@patsnap.com