CNN-deep-learning-based bridge-crack detecting and positioning method
A technology of deep learning and positioning method, which is applied in the field of image processing and computer vision, can solve problems such as crack detection and positioning in color images, and achieve good generalization ability, strong adaptability, and fast processing speed
- Summary
- Abstract
- Description
- Claims
- Application Information
AI Technical Summary
Problems solved by technology
Method used
Image
Examples
Embodiment 1
[0057] The main task of the invention is to detect and locate bridge cracks for pictures of bridge cracks with colors, different background textures and different materials. combine Figure 1-Figure 2 , a bridge crack detection and positioning method based on CNN deep learning method, comprising the following steps:
[0058] The first step is to use the camera sensor to collect a certain number of bridge crack pictures, and normalize all the pictures to 1024*1024 resolution pictures;
[0059] In the second step, use a fixed-size window of W*H to slide on the bridge crack image without overlapping, and at the same time, use the small slice of the bridge crack image covered by the sliding window as a ROI region of interest. Among them, the small slice image containing the bridge background is called the bridge background surfel, and the small slice containing the bridge crack is called the bridge crack surfel. The specific process is shown in the following formula:
[0060] im...
Embodiment 2
[0086] Refer below Figure 1-Figure 4 , using specific data to describe the present invention in detail:
[0087] The first step is to use image acquisition equipment to collect five kinds of bridge crack pictures with different background textures and different materials. The total number of collected pictures is 2000, and all pictures are normalized into pictures with a resolution of 1024*1024;
[0088] In the second step, the 2000 pictures are divided into 2 data sets, artificial amplification data set and test data set, each data set has 1000 pictures;
[0089] The third step is to use a W*H fixed-size window to slide non-overlapping 1000 pictures in the artificially amplified data set, and at the same time, use the small slice of the bridge crack picture covered by the sliding window as a ROI region of interest. Among them, the small slice image containing the bridge background is called the bridge background surfel, and the small slice containing the bridge crack is cal...
Embodiment 3
[0107] This embodiment discloses a method for constructing a DBCC classification model based on CNN deep learning, comprising the following steps:
[0108] (1) Convolute and sum the input original picture with all the convolution kernels in the first convolutional layer in a convolutional manner to obtain the feature map of the first convolutional layer;
[0109] (2) Add a Relu activation function after the first convolutional layer;
[0110] (3) After the first convolutional layer, add a local response value normalization layer for picture brightness correction, and the local response value normalization layer improves the recognition effect of the network;
[0111] (4) Downsample the feature map of the first convolutional layer in the first pooling layer, reduce the resolution and select excellent features as the feature map of the first pooling layer;
[0112] (5) On the second convolutional layer, the feature map of the first pooling layer and all the convolution kernels ...
PUM
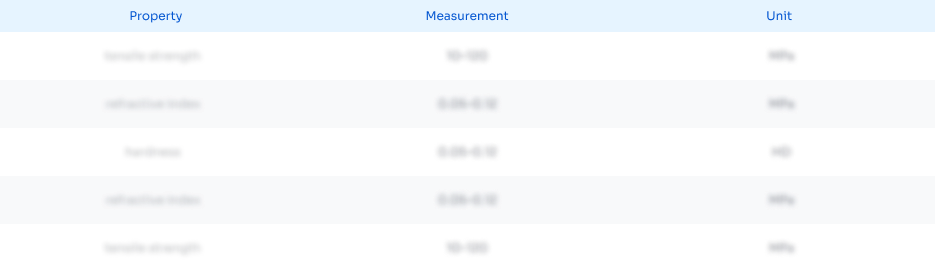
Abstract
Description
Claims
Application Information
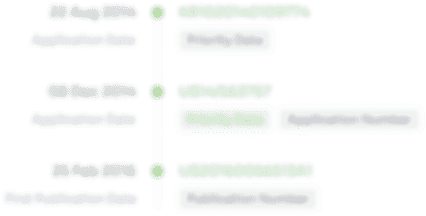
- R&D Engineer
- R&D Manager
- IP Professional
- Industry Leading Data Capabilities
- Powerful AI technology
- Patent DNA Extraction
Browse by: Latest US Patents, China's latest patents, Technical Efficacy Thesaurus, Application Domain, Technology Topic, Popular Technical Reports.
© 2024 PatSnap. All rights reserved.Legal|Privacy policy|Modern Slavery Act Transparency Statement|Sitemap|About US| Contact US: help@patsnap.com