Road surface abandoned object detection method based on deep convolutional network
A technology of deep convolution and detection method, which is applied in the field of detection of road residues based on deep convolutional neural network, can solve the problems of inability to effectively manage existing video resources, and achieves a low-cost, low-cost hardware investment. Effect
- Summary
- Abstract
- Description
- Claims
- Application Information
AI Technical Summary
Problems solved by technology
Method used
Image
Examples
Embodiment Construction
[0028] The invention introduces deep learning into road event recognition and improves it, which can significantly improve the accuracy of road event recognition. Considering that the background modeling method is not suitable for static object detection, and the pavement remnants are difficult to use the prior model to construct the training set. The invention establishes the detection of road remnants based on deep convolutional neural network Deep-CNN. The detection point of the mobile terminal is a road camera, and the detection point of the mobile terminal obtains image information through the camera, and the CNN neural network is used to analyze the obtained image. The present invention Divide the pavement ROI area into multiple networks, build a pavement-non-pavement recognition model, and reversely identify static targets such as highway pavement remnants and road spills through non-pavement grids.
[0029] The present invention builds the layered road event recognitio...
PUM
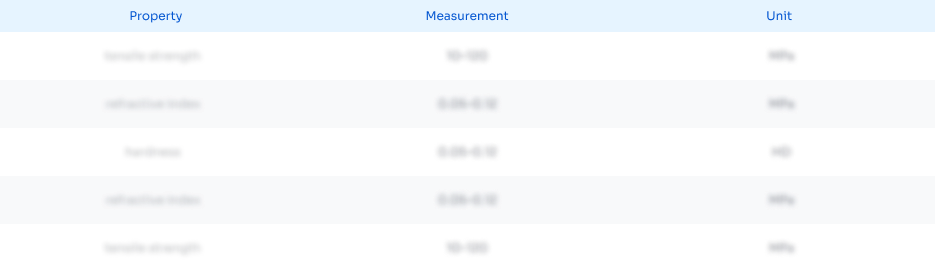
Abstract
Description
Claims
Application Information
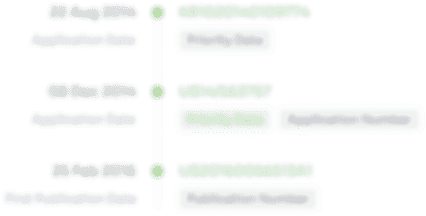
- R&D Engineer
- R&D Manager
- IP Professional
- Industry Leading Data Capabilities
- Powerful AI technology
- Patent DNA Extraction
Browse by: Latest US Patents, China's latest patents, Technical Efficacy Thesaurus, Application Domain, Technology Topic, Popular Technical Reports.
© 2024 PatSnap. All rights reserved.Legal|Privacy policy|Modern Slavery Act Transparency Statement|Sitemap|About US| Contact US: help@patsnap.com