Data feature-based deep neural network self-training method
A deep neural network and data feature technology, applied in neural learning methods, biological neural network models, etc., can solve problems such as lack of wide applicability, and achieve the effects of avoiding model adjustment, high test accuracy, and high prediction accuracy.
- Summary
- Abstract
- Description
- Claims
- Application Information
AI Technical Summary
Problems solved by technology
Method used
Image
Examples
Embodiment
[0042] In order to verify the feasibility and effectiveness of this method, the following experiments are used to specifically illustrate:
[0043] Taking two-dimensional data as an example, an image classification problem is designed for this method. When several image classification sample libraries are known, use this method to build a neural network model, and then test the classification accuracy of the model.
[0044] Select a number of different sample libraries as the sample set of the trainer, including ORL Faces face library and several sample libraries from UCI Machine Learning Repository. Candidate parameters are: network depth (the number of hidden layers) is 1, 2, 3; the number of convolution kernels is 6, 10, 12, 16, 20, 32, 64 (the number of nodes in the latter layer is more than that of the previous one Layer); the size of the convolution kernel is 3x3, 5x5, 7x7; the gradient method is stochastic gradient descent, Momentum, Adam; the initial training step size is ...
PUM
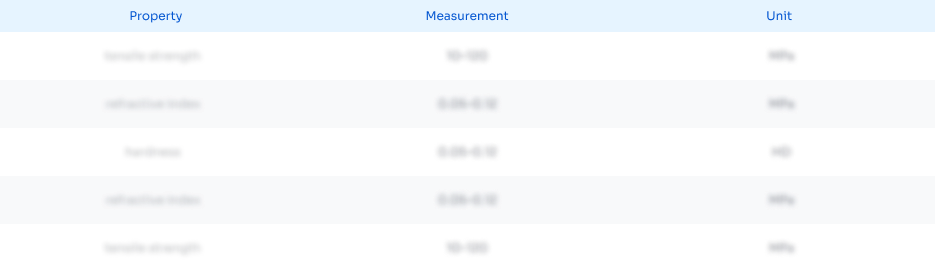
Abstract
Description
Claims
Application Information
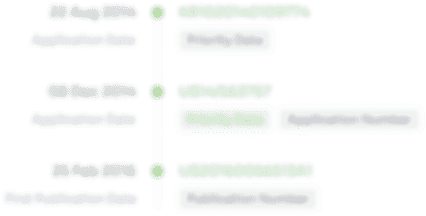
- R&D Engineer
- R&D Manager
- IP Professional
- Industry Leading Data Capabilities
- Powerful AI technology
- Patent DNA Extraction
Browse by: Latest US Patents, China's latest patents, Technical Efficacy Thesaurus, Application Domain, Technology Topic, Popular Technical Reports.
© 2024 PatSnap. All rights reserved.Legal|Privacy policy|Modern Slavery Act Transparency Statement|Sitemap|About US| Contact US: help@patsnap.com