Medical image classification method for combining deep characteristic extraction and shallow characteristic extraction
A deep feature, medical image technology, applied in character and pattern recognition, instruments, computer parts, etc., can solve problems such as low accuracy rate and poor classification algorithm effect, achieve high automatic classification, overcome the effect of poor effect
- Summary
- Abstract
- Description
- Claims
- Application Information
AI Technical Summary
Problems solved by technology
Method used
Image
Examples
Embodiment Construction
[0024] Now in conjunction with embodiment, accompanying drawing, the present invention will be further described:
[0025] The present invention provides an innovative method for simultaneously performing feature extraction based on deep convolutional neural network and heuristic feature extraction guided by domain knowledge, and combining the obtained two types of features to train a classifier to realize medical image classification.
[0026] This method extracts several small image blocks from each medical image, and the category of each image block is the category of the image it is in, so that the classification problem based on the image is transformed into the classification problem based on the image block. First, use the image blocks extracted from all training images to train a deep convolutional neural network model using the stochastic gradient descent method, and select the output of the fully connected layer of the network as the descriptive features of the corres...
PUM
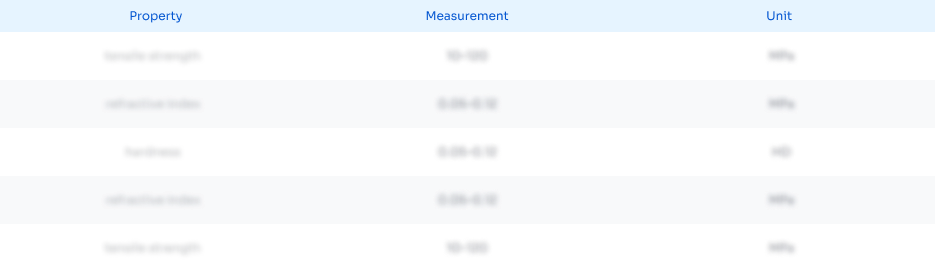
Abstract
Description
Claims
Application Information
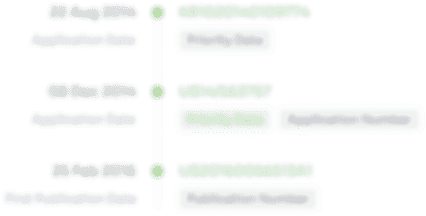
- R&D Engineer
- R&D Manager
- IP Professional
- Industry Leading Data Capabilities
- Powerful AI technology
- Patent DNA Extraction
Browse by: Latest US Patents, China's latest patents, Technical Efficacy Thesaurus, Application Domain, Technology Topic, Popular Technical Reports.
© 2024 PatSnap. All rights reserved.Legal|Privacy policy|Modern Slavery Act Transparency Statement|Sitemap|About US| Contact US: help@patsnap.com