A Method of Selecting Hyperspectral Characteristic Variables
A feature variable and hyperspectral technology, applied in the field of image processing, can solve problems such as large running time, large amount of calculation, and no good solution to many types of problems, and achieve the effect of reducing complexity and high accuracy
- Summary
- Abstract
- Description
- Claims
- Application Information
AI Technical Summary
Problems solved by technology
Method used
Image
Examples
Embodiment Construction
[0025] The present invention will be further described below in conjunction with the accompanying drawings and specific embodiments.
[0026] For the convenience of expression, the following definitions are now made: Consider M pairs of training sets S={i ,Y i >},i∈[1,M];X i (∈R N ): i-th sample eigenvector (that is, reflectivity on each band (dimension), composed of reflectivity vector); R: real number set; R N : Nth power set of the real number set R; N: feature dimension; Y i :X i The class label of , for the second class problem, Y i ∈{-1,1}, for class k problems (k>2), suppose Y i ∈[1,k]. The purpose of using support vector machine classification:
[0027] Find a hyperplane (decision plane) that maximizes the distance between it and the nearest sample of the two classes. The decision plane is defined as f(X)=ω T X+b, where ω=[ω 1 , ω 2 ,...,ω i ,...,ω N ] T is the correlation coefficient vector, where ω i is the coefficient corresponding to the i-th dimensi...
PUM
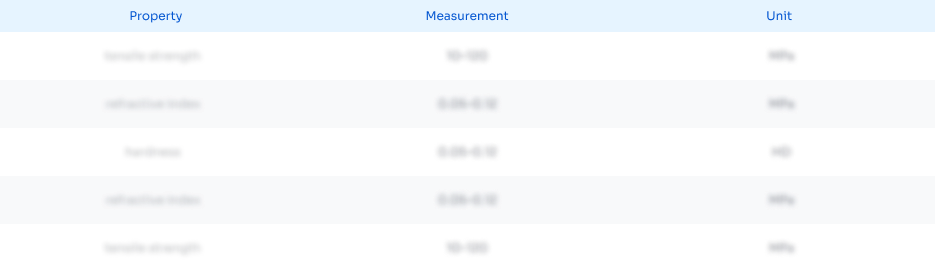
Abstract
Description
Claims
Application Information
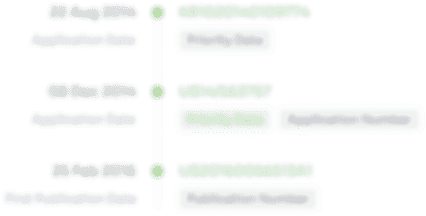
- R&D Engineer
- R&D Manager
- IP Professional
- Industry Leading Data Capabilities
- Powerful AI technology
- Patent DNA Extraction
Browse by: Latest US Patents, China's latest patents, Technical Efficacy Thesaurus, Application Domain, Technology Topic, Popular Technical Reports.
© 2024 PatSnap. All rights reserved.Legal|Privacy policy|Modern Slavery Act Transparency Statement|Sitemap|About US| Contact US: help@patsnap.com