Hyperspectral image classification method based on multi-scale spatial-spectral feature joint learning
A technology of hyperspectral image and classification method, applied in the field of hyperspectral image classification, can solve the problems of single feature scale and limited receptive field, and achieve the effect of improving classification accuracy, ensuring classification performance, and speeding up training speed.
- Summary
- Abstract
- Description
- Claims
- Application Information
AI Technical Summary
Problems solved by technology
Method used
Image
Examples
Embodiment Construction
[0029] In order to make those skilled in the art better understand the solutions of the present application, the following will clearly and completely describe the technical solutions in the embodiments of the present application with reference to the accompanying drawings in the embodiments of the present application.
[0030] The present invention provides a method for classifying hyperspectral images based on joint learning of multi-scale spatial spectral features. A network model based on multi-scale spectral-spatial features is used to classify ground object categories contained in hyperspectral images. figure 1 As shown, the network model includes: a multi-scale spectral feature extraction module, a multi-scale spatial feature extraction module, a spectral-spatial feature fusion module, and a spectral-spatial feature classification module; the functions of each module are as follows:
[0031] The multi-scale spectral feature extraction module is used to input the hyperspe...
PUM
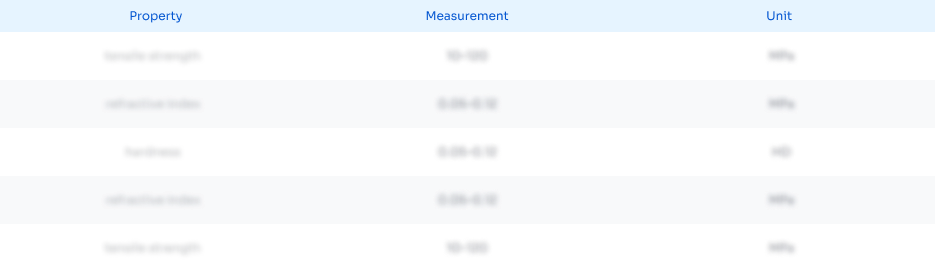
Abstract
Description
Claims
Application Information
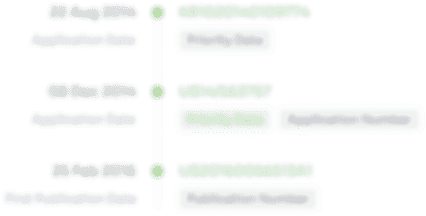
- R&D Engineer
- R&D Manager
- IP Professional
- Industry Leading Data Capabilities
- Powerful AI technology
- Patent DNA Extraction
Browse by: Latest US Patents, China's latest patents, Technical Efficacy Thesaurus, Application Domain, Technology Topic, Popular Technical Reports.
© 2024 PatSnap. All rights reserved.Legal|Privacy policy|Modern Slavery Act Transparency Statement|Sitemap|About US| Contact US: help@patsnap.com