Heterogeneous GPU distribution system and method for multiple deep learning tasks in distributed environment
A distributed environment and learning task technology, applied in the field of heterogeneous GPU allocation system, can solve the problems of not considering task characteristics and requirements, low GPU utilization rate, low execution efficiency of multiple deep learning tasks, etc., to save waiting for results Time, the effect of improving execution efficiency
- Summary
- Abstract
- Description
- Claims
- Application Information
AI Technical Summary
Problems solved by technology
Method used
Image
Examples
Embodiment
[0062] According to the current heterogeneous GPU configuration, whether the GPU's memory capacity can hold the batch data volume of multiple tasks at the same time, and the execution efficiency of deep learning training for each task, etc. A heterogeneous GPU allocation method for multiple deep learning tasks in a multi-level environment, including the following steps:
[0063] S1, initialization of multiple deep learning training tasks; such as figure 2 It shows the data flow of multi-task deep learning training in a heterogeneous environment. The heterogeneity shown in the present invention is only different from the GPU. The original data stored in the bottom layer is transmitted to the DRAM cache of the heterogeneous environment. The time is roughly the same, and the present invention needs to optimize how the batch data for the memory cache is better distributed to the corresponding GPU. When the multi-task deep learning is initialized, the GPU Profile module collects ...
PUM
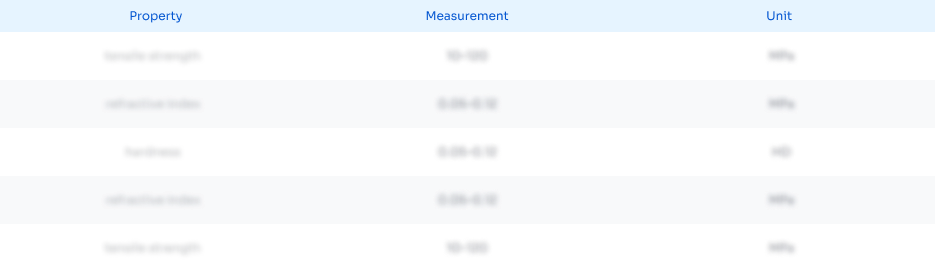
Abstract
Description
Claims
Application Information
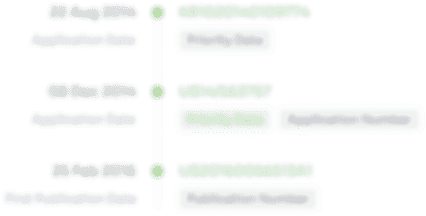
- R&D Engineer
- R&D Manager
- IP Professional
- Industry Leading Data Capabilities
- Powerful AI technology
- Patent DNA Extraction
Browse by: Latest US Patents, China's latest patents, Technical Efficacy Thesaurus, Application Domain, Technology Topic, Popular Technical Reports.
© 2024 PatSnap. All rights reserved.Legal|Privacy policy|Modern Slavery Act Transparency Statement|Sitemap|About US| Contact US: help@patsnap.com