Method for robustly classifying pictures by using sparse network based on retention dynamics process
A dynamic and sparse technology, applied in neural learning methods, biological neural network models, instruments, etc., can solve the problem that fine-tuning is not necessarily useful, and achieve the effect of speeding up inference, reducing occupancy, and excellent adversarial robustness.
- Summary
- Abstract
- Description
- Claims
- Application Information
AI Technical Summary
Problems solved by technology
Method used
Image
Examples
Embodiment Construction
[0072] The task objective of the present invention is to use a sparse network and train it adversarially to obtain an adversarially robust image classifier. Each step of the present invention will be described below according to examples.
[0073] Dataset introduction: The dataset is CIFAR-10, see figure 1 , contains a total of 10 categories of RGB color images: airplanes, cars, birds, cats, deer, dogs, frogs, horses, boats, and trucks. The size of the images is 32×32, and there are a total of 50,000 training images and 10,000 test images in the dataset.
[0074] Network introduction: The network structure adopts the classic convolutional neural network VGG-16, see figure 2 , the network is mainly composed of 5 convolutional layers and 3 fully connected layers, each convolutional layer is followed by a pooling layer to expand the receptive field, and each fully connected layer is followed by a dropout layer to reduce overfitting , and finally use a softmax layer for normal...
PUM
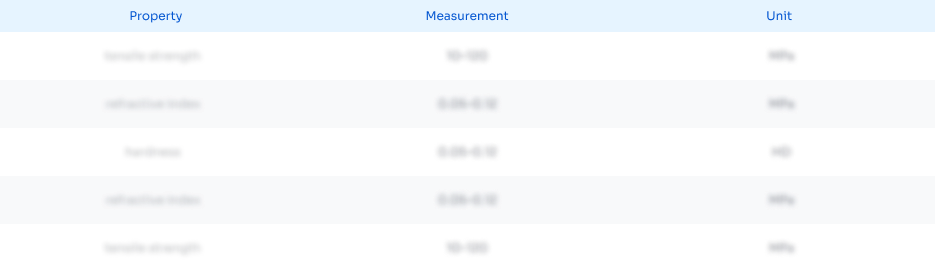
Abstract
Description
Claims
Application Information
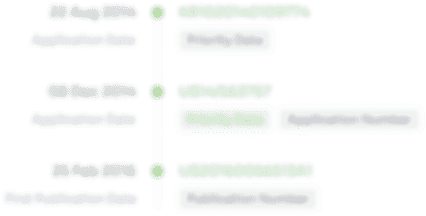
- R&D Engineer
- R&D Manager
- IP Professional
- Industry Leading Data Capabilities
- Powerful AI technology
- Patent DNA Extraction
Browse by: Latest US Patents, China's latest patents, Technical Efficacy Thesaurus, Application Domain, Technology Topic, Popular Technical Reports.
© 2024 PatSnap. All rights reserved.Legal|Privacy policy|Modern Slavery Act Transparency Statement|Sitemap|About US| Contact US: help@patsnap.com