Atmospheric ozone concentration prediction method based on mixed CNN-Transformer model
A technology of ozone concentration and prediction method, applied in the direction of prediction, biological neural network model, data processing application, etc., to achieve the effect of making up for the lack of extraction ability
- Summary
- Abstract
- Description
- Claims
- Application Information
AI Technical Summary
Problems solved by technology
Method used
Image
Examples
Embodiment Construction
[0037]The following will clearly and completely describe the technical solutions in the embodiments of the present invention with reference to the drawings in the embodiments of the present invention. Apparently, the described embodiments are only some of the embodiments of the present invention, but not all of them. Based on the embodiments of the present invention, all other embodiments obtained by persons of ordinary skill in the art without making creative efforts belong to the protection scope of the present invention.
[0038] The purpose of the present invention is to provide a method for predicting atmospheric ozone concentration based on a hybrid CNN-Transformer model, which solves the problems that the prediction accuracy of traditional prediction models is not high and the traditional Transformer model has insufficient ability to extract multi-dimensional features, based on the historical air quality of Beijing monitoring sites Data and meteorological data, combined...
PUM
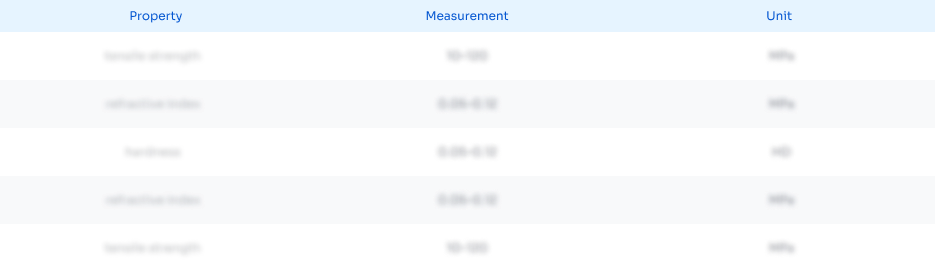
Abstract
Description
Claims
Application Information
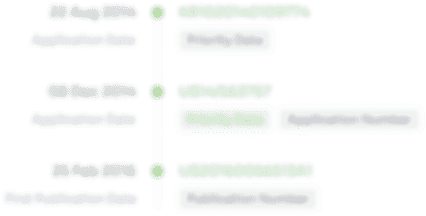
- R&D Engineer
- R&D Manager
- IP Professional
- Industry Leading Data Capabilities
- Powerful AI technology
- Patent DNA Extraction
Browse by: Latest US Patents, China's latest patents, Technical Efficacy Thesaurus, Application Domain, Technology Topic, Popular Technical Reports.
© 2024 PatSnap. All rights reserved.Legal|Privacy policy|Modern Slavery Act Transparency Statement|Sitemap|About US| Contact US: help@patsnap.com