Fiberboard quality classification method based on machine vision
A quality classification and machine vision technology, applied in the direction of instruments, image analysis, computer parts, etc., can solve the problems of threshold segmentation to obtain the fiberboard surface, no defect direction, and morphological feature analysis, so as to improve the timeliness of repair, The effect of reducing labor intensity and accurate test results
- Summary
- Abstract
- Description
- Claims
- Application Information
AI Technical Summary
Problems solved by technology
Method used
Image
Examples
Embodiment 1
[0053]A kind of embodiment of the fiberboard quality classification method based on machine vision of the present invention, as figure 1 shown, including:
[0054] S101. Obtaining a grayscale image of the surface of the fiberboard
[0055] Use machine vision to collect the surface image of the fiberboard, perform semantic segmentation on the collected image to remove background interference, and then multiply the image after semantic segmentation with the collected image and perform grayscale processing to facilitate subsequent operations on the features in the image extraction and analysis.
[0056] S102. Obtain the sliding window area corresponding to each pixel
[0057] Taking each pixel in the grayscale image of the fiberboard surface as the center, the sliding window processing is performed to obtain the sliding window area corresponding to each pixel. The gray value difference of the pixel points of the same fiber in the defective fiberboard is small, so the pixel Poi...
Embodiment 2
[0073] A kind of embodiment of the fiberboard quality classification method based on machine vision of the present invention, as figure 2 shown, including:
[0074] S201. Obtain a grayscale image of the fiberboard surface
[0075] Use machine vision to collect the surface image of the fiberboard, perform semantic segmentation on the collected image to remove background interference, and then multiply the image after semantic segmentation with the collected image and perform grayscale processing to facilitate subsequent operations on the features in the image extraction and analysis.
[0076] Arrange the camera, collect the image of the fiberboard, and use DNN semantic segmentation to identify and segment the target area in the image. The specific process is as follows:
[0077] 1) The data set used is a product image data set collected from a top view, and the styles of fiberboards are various.
[0078] 2) The pixels that need to be segmented are divided into two categorie...
PUM
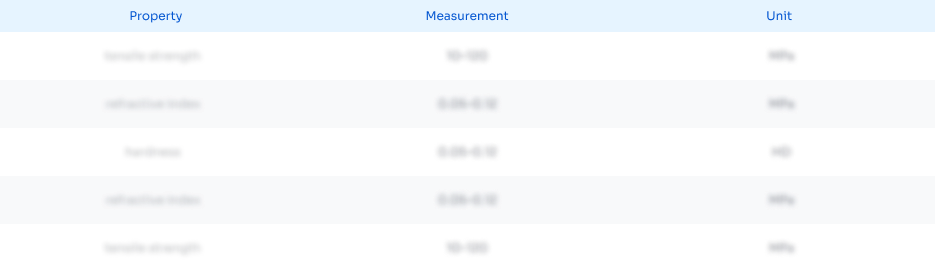
Abstract
Description
Claims
Application Information
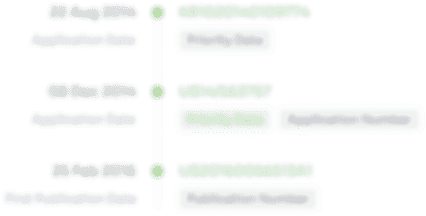
- R&D Engineer
- R&D Manager
- IP Professional
- Industry Leading Data Capabilities
- Powerful AI technology
- Patent DNA Extraction
Browse by: Latest US Patents, China's latest patents, Technical Efficacy Thesaurus, Application Domain, Technology Topic, Popular Technical Reports.
© 2024 PatSnap. All rights reserved.Legal|Privacy policy|Modern Slavery Act Transparency Statement|Sitemap|About US| Contact US: help@patsnap.com