Indicator diagram fault diagnosis method based on generative adversarial neural network
A neural network and fault diagnosis technology, applied in the direction of biological neural network model, neural learning method, neural architecture, etc., can solve the problem of high false positive/missing rate of faults, improve specific recognition ability, reduce false positives/missing negatives the effect of the situation
- Summary
- Abstract
- Description
- Claims
- Application Information
AI Technical Summary
Problems solved by technology
Method used
Image
Examples
Embodiment
[0171] This embodiment is based on the field data of an oil field, and there are 14628 pieces of dynamometer working condition data in total.
[0172] First, according to the distribution of the number of samples, the anti-neural network GAN is used to generate samples for failures such as continuous pumping and spraying, pump leakage, and other explanations, and 200 new samples are generated for each. This embodiment does not involve the optimization of hyperparameters, so the verification set data does not interfere with the model, so there is no need to divide the test set separately, only the training set and the verification set need to be divided. Among them: 80% of the samples are used as the training set, and 20% of the samples are used as the verification set.
[0173] Then, establish the Xgboost classifier model according to the above step 5, and perform fault diagnosis on the samples.
[0174] Finally, the accuracy and recall rate of the verification set are calcul...
PUM
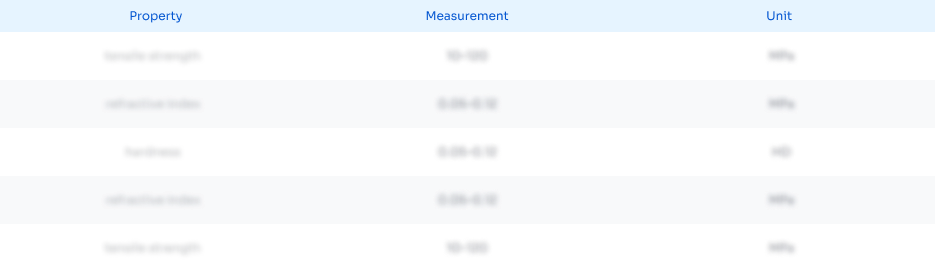
Abstract
Description
Claims
Application Information
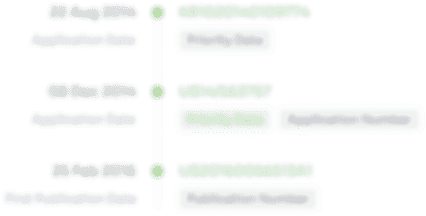
- Generate Ideas
- Intellectual Property
- Life Sciences
- Materials
- Tech Scout
- Unparalleled Data Quality
- Higher Quality Content
- 60% Fewer Hallucinations
Browse by: Latest US Patents, China's latest patents, Technical Efficacy Thesaurus, Application Domain, Technology Topic, Popular Technical Reports.
© 2025 PatSnap. All rights reserved.Legal|Privacy policy|Modern Slavery Act Transparency Statement|Sitemap|About US| Contact US: help@patsnap.com