Queuing network model training method, queuing optimization method, equipment and medium
A queuing network and training method technology, applied in the field of queuing optimization processing, can solve problems such as calculation errors, non-compliance, and inability to automate, and achieve high prediction accuracy
- Summary
- Abstract
- Description
- Claims
- Application Information
AI Technical Summary
Problems solved by technology
Method used
Image
Examples
Embodiment 1
[0045] A training method for a queuing network model, such as figure 1 As shown, the training method includes:
[0046] Step 11, obtaining the real-time queuing network diagram; the real-time queuing network diagram includes a plurality of real-time queuing nodes and the node characteristics of each real-time queuing node;
[0047] Among them, after the on-site data is obtained, the data is cleaned, including standardization, normalization, and regularization processing, for subsequent training. The data includes but is not limited to the number of nodes (each node is a queuing point), node Adjacency matrix (generally a fully connected graph, you can adjust whether the edge exists according to the site situation) and node characteristics (including queuing item, gender, age, number of nodes in the queue, processor ID, processing item number, other characteristics of queuing people, node other characteristics and start queue times).
[0048] Step 12, inputting the real-time q...
Embodiment 2
[0067] A queuing optimization method such as Figure 4 As shown, the optimization method includes:
[0068] Step 41, obtaining the queuing network map to be processed; the queuing network map to be processed includes a plurality of nodes to be processed and the node characteristics of each node to be processed;
[0069] Step 42, input the queuing network diagram to be processed into the queuing network model obtained by the training method of the queuing network model in embodiment 1, and output the optimized queuing network diagram;
[0070] Wherein, the optimized queuing network graph includes optimized features of each node to be processed.
[0071] Step 43 , generating an access sequence of each node to be processed based on the optimization feature.
[0072] Among them, the feature of each node is directly output by the decoder according to the one-hot format, which is used to identify the classification of the node.
[0073] Step 44: Process the optimized features of ...
Embodiment 3
[0082] An electronic device, comprising a memory, a processor, and a computer program stored on the memory and operable on the processor, wherein the processor implements the queuing network model described in Embodiment 1 when executing the computer program training method, and / or, the queuing optimization method described in Embodiment 2 is implemented when the computer program is executed.
[0083] Figure 5 A schematic structural diagram of an electronic device provided in this embodiment. Figure 5 A block diagram of an exemplary electronic device 90 suitable for use in implementing embodiments of the invention is shown. Figure 5 The electronic device 90 shown is only an example, and should not limit the functions and scope of use of the embodiments of the present invention.
[0084] Such as Figure 5 As shown, electronic device 90 may take the form of a general-purpose computing device, which may be a server device, for example. Components of the electronic device 9...
PUM
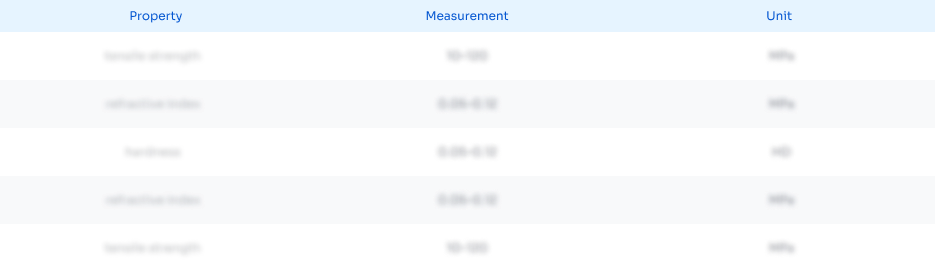
Abstract
Description
Claims
Application Information
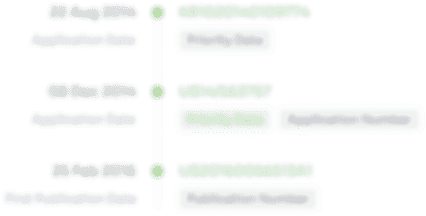
- R&D
- Intellectual Property
- Life Sciences
- Materials
- Tech Scout
- Unparalleled Data Quality
- Higher Quality Content
- 60% Fewer Hallucinations
Browse by: Latest US Patents, China's latest patents, Technical Efficacy Thesaurus, Application Domain, Technology Topic, Popular Technical Reports.
© 2025 PatSnap. All rights reserved.Legal|Privacy policy|Modern Slavery Act Transparency Statement|Sitemap|About US| Contact US: help@patsnap.com