Classification adversarial fraud detection method based on class clear representation
A detection method and adversarial technology, applied in the field of data processing, can solve the problems of fraudulent behavior, such as large data volume and data dimension, difficult data acquisition, and lack of negative samples.
- Summary
- Abstract
- Description
- Claims
- Application Information
AI Technical Summary
Problems solved by technology
Method used
Image
Examples
Embodiment Construction
[0056] In order to better understand the technical solution, the method of the present invention will be described in detail below in conjunction with the accompanying drawings.
[0057] A classification deep learning method refers to the use of deep learning methods or models to learn when there is only one class (in this method, only normal behavior data), and finally achieve the purpose of classification.
[0058] The following is an introduction to the models commonly used in a classification deep learning method:
[0059] (1) Autoencoder
[0060] In 1986, Rumelhart proposed the concept of autoencoder and used it to process high-dimensional complex data, which promoted the development of neural networks. Autoencoder neural network is an unsupervised learning algorithm that is often used to reduce the dimensionality of data. One of its most common applications is anomaly detection. After data dimensionality reduction, key features are extracted, making classifiers easier t...
PUM
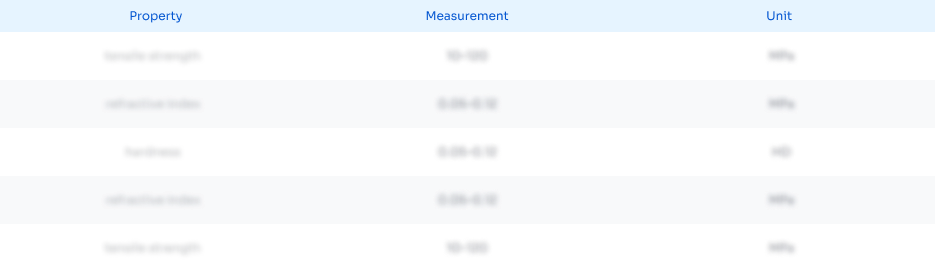
Abstract
Description
Claims
Application Information
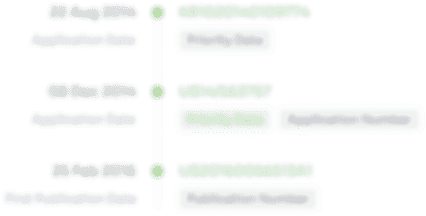
- R&D Engineer
- R&D Manager
- IP Professional
- Industry Leading Data Capabilities
- Powerful AI technology
- Patent DNA Extraction
Browse by: Latest US Patents, China's latest patents, Technical Efficacy Thesaurus, Application Domain, Technology Topic, Popular Technical Reports.
© 2024 PatSnap. All rights reserved.Legal|Privacy policy|Modern Slavery Act Transparency Statement|Sitemap|About US| Contact US: help@patsnap.com