An abnormal detection method for power Internet of things equipment based on graph neural network
A technology for power Internet of Things and equipment anomalies, applied in the direction of neural learning methods, biological neural network models, neural architectures, etc., can solve the problem of low accuracy, failure to use equipment space characteristics, failure to extract power Internet of Things equipment space characteristics, etc. problems, to achieve the effect of enhancing rationality and reducing false positives
- Summary
- Abstract
- Description
- Claims
- Application Information
AI Technical Summary
Problems solved by technology
Method used
Image
Examples
Embodiment Construction
[0063] In order to understand the above-mentioned purpose, features and advantages of the present invention more clearly, the present invention will be further described in detail below in conjunction with the accompanying drawings and specific embodiments. In the following description, many specific details are set forth in order to fully understand the present invention, but the present invention can also be implemented in other ways different from those described here, therefore, the present invention is not limited to the specific embodiments disclosed below limit.
[0064] Unless otherwise defined, the technical terms or scientific terms used herein shall have the common meanings understood by those having ordinary skill in the field to which this application relates. "First", "second" and similar words used in the specification and claims of this patent application do not indicate any sequence, quantity or importance, but are only used to distinguish different components...
PUM
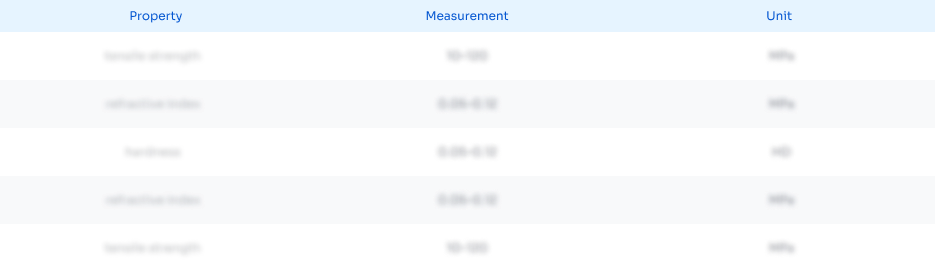
Abstract
Description
Claims
Application Information
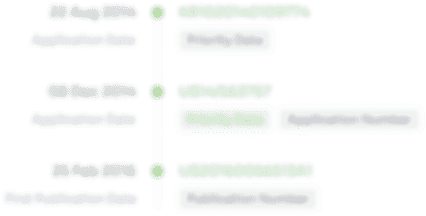
- R&D Engineer
- R&D Manager
- IP Professional
- Industry Leading Data Capabilities
- Powerful AI technology
- Patent DNA Extraction
Browse by: Latest US Patents, China's latest patents, Technical Efficacy Thesaurus, Application Domain, Technology Topic, Popular Technical Reports.
© 2024 PatSnap. All rights reserved.Legal|Privacy policy|Modern Slavery Act Transparency Statement|Sitemap|About US| Contact US: help@patsnap.com