Deep learning model compression method based on decision boundary
A technology of deep learning and compression methods, applied in neural learning methods, biological neural network models, neural architectures, etc., can solve problems such as consuming large computing power, and achieve the effect of model compression
- Summary
- Abstract
- Description
- Claims
- Application Information
AI Technical Summary
Problems solved by technology
Method used
Image
Examples
Embodiment Construction
[0040] The technical solutions in the embodiments of the present invention will be clearly and completely described below in conjunction with the accompanying drawings in the embodiments of the present invention. Obviously, the described embodiments are only some, not all, embodiments of the present invention. Based on the embodiments of the present invention, all other embodiments obtained by persons of ordinary skill in the art without making creative efforts belong to the protection scope of the present invention.
[0041] The present invention proposes a method for compressing a deep learning model based on a decision boundary, and the method for compressing a deep learning model based on a decision boundary includes the following steps:
[0042] Step 1. Perform feature mapping;
[0043] Step 2, performing piecewise linearization of the activation function;
[0044] Step 3: Calculate the sub-decision area: calculate the sub-decision area of the fully connected layer;
[...
PUM
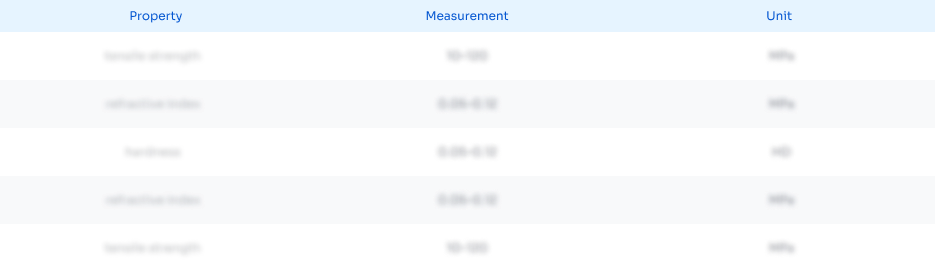
Abstract
Description
Claims
Application Information
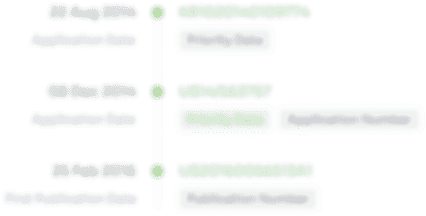
- R&D Engineer
- R&D Manager
- IP Professional
- Industry Leading Data Capabilities
- Powerful AI technology
- Patent DNA Extraction
Browse by: Latest US Patents, China's latest patents, Technical Efficacy Thesaurus, Application Domain, Technology Topic, Popular Technical Reports.
© 2024 PatSnap. All rights reserved.Legal|Privacy policy|Modern Slavery Act Transparency Statement|Sitemap|About US| Contact US: help@patsnap.com