Systems and methods for karyotyping by sequencing
A karyotype and model technology, applied in the field of karyotype analysis systems and methods through sequencing, can solve problems such as labor-intensive, limited processing capacity and efficiency, and liquid cancer difficulties
- Summary
- Abstract
- Description
- Claims
- Application Information
AI Technical Summary
Problems solved by technology
Method used
Image
Examples
example 1
[0568] Example 1: Genotyping Human Structural Variants of Known Significance
[0569] In one embodiment ( Figure 4 A-C), Likelihood model classifiers were created and used to identify variants of known clinical significance in human samples. A likelihood model classifier was trained using Hi-C data from both mock and biological samples, reflecting the structural changes present in the samples. Detect variants with a likelihood model classifier by providing Hi-C data from clinical or research samples outside the training set. The likelihood model classifier represents all variants as bounding rectangles encoding the start and end positions (in genomic bands) of structural variants, with markers. Markers can describe the nature of the variation, such as balanced or unbalanced translocations, inversions or insertions, deletions or repeat expansions. The list of variants with known clinical significance is also input into the likelihood model classifier, where the entire set o...
example 2
[0586] Example 2: Detecting and Annotating All Structural Variations in an Organism Using Convolutional Neural Networks (CNNs)
[0587] In another KBS implementation ( Figure 5 A-C), An ensemble of deep learning models is created and used to identify any structural variation in an organism, and to assign a possible action, explanation, or meaning to the variation based on known clinical or biological data. This implementation includes two machine learning models.
[0588]In this example, the first machine learning model is a convolutional neural network (CNN), which receives a contact matrix as input. This matrix can be averaged to a resolution such that it would be computationally feasible to feed the matrix into a CNN (e.g. each cell in the matrix represents 1,000,000 base pairs), or a continuously scalable data structure (e.g. for the first A KD-tree data structure described by the main application). The first machine learning model detects regions of the contact matrix...
example 3
[0608] Example 3: Detecting and Annotating All Structural Variations in an Organism Using an Edge Detection Algorithm
[0609] This is a multifaceted approach that represents the Hi-C connectivity density between a pair of chromosomes as pixels in an image, and then uses a series of image processing techniques and novel algorithms to identify translocation bounding boxes and insertion points. Preprocessing steps including global normalization, global thresholding, and image-by-image denoising are applied to the image, and then three edge / corner detection algorithms / modules (Harris corner method, Roberts cross, Hough transform) are used to Larger changes in signal intensity gradients are identified and those signals are converted to bounding boxes (structural variation calls). Additional filters were applied to remove false positives, including a novel recursive algorithm for eliminating false detections close to the diagonal of images within the contig.
[0610] False positiv...
PUM
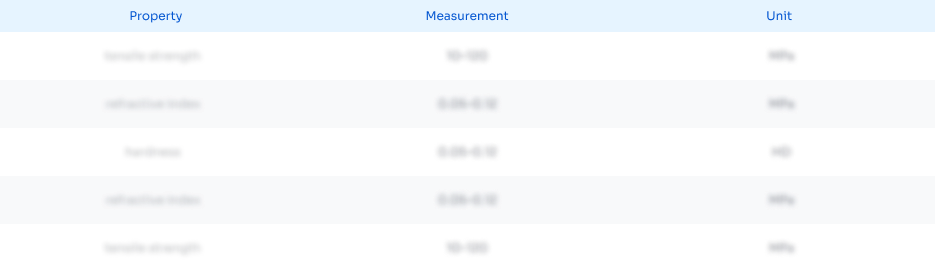
Abstract
Description
Claims
Application Information
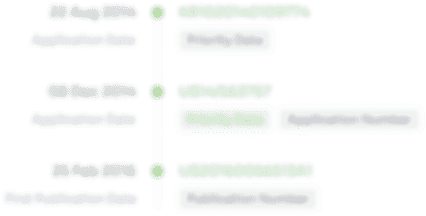
- R&D Engineer
- R&D Manager
- IP Professional
- Industry Leading Data Capabilities
- Powerful AI technology
- Patent DNA Extraction
Browse by: Latest US Patents, China's latest patents, Technical Efficacy Thesaurus, Application Domain, Technology Topic.
© 2024 PatSnap. All rights reserved.Legal|Privacy policy|Modern Slavery Act Transparency Statement|Sitemap