Molecular screening method based on deep learning technology qualitative and quantitative model
A quantitative model and deep learning technology, applied in the fields of material crossover, deep learning and physics, and chemistry, to achieve high-precision molecular prediction, solve limitations, and accurately screen
- Summary
- Abstract
- Description
- Claims
- Application Information
AI Technical Summary
Problems solved by technology
Method used
Image
Examples
Embodiment 1
[0054] A molecular screening method based on qualitative and quantitative models of deep learning technology, the realization process is as follows figure 1 shown. It includes the following steps: construction and preprocessing of molecular data sets, feature engineering of data sets, construction and training of qualitative and quantitative models, deployment of model prediction and screening.
[0055] 1. The construction and preprocessing of molecular data sets, specifically including the following steps:
[0056] (1) Convert the molecular structure formula to SMILES. First obtain the molecule used for training the model and its molecular structural formula, according to the SMILES conversion rule developed by DAVID WEININGER in 1988, the perovskite FAPbI 3 Taking additives in light-emitting diodes as an example, the structural formula of each additive molecule is converted into SMILES.
[0057] (2) Transform SMILES into qualitative and quantitative descriptors, respectiv...
Embodiment 2
[0069] When constructing a qualitative and quantitative model, FP2 fingerprints and 65 attributes are selected for joint input, and a five-layer network is set in the model such as Figure 4 As shown, the specific construction and training of qualitative and quantitative models refer to the description in Example 1.
[0070] At this time, the verification accuracy of the qualitative and quantitative model reaches 85.71%. Compared with the FP2 fingerprint input in the DNN model, the verification accuracy can only reach 75.00%, and the accuracy of the qualitative and quantitative model has increased by 10.71%. The contrast accuracy at a glance is as follows Figure 8 shown.
Embodiment 3
[0072] When building a qualitative and quantitative model, the joint input of MACCS fingerprint and 65 attributes is selected, and a five-layer network is set in the model such as Figure 4 As shown, the specific construction and training of qualitative and quantitative models refer to the description in Example 1.
[0073] At this time, the verification accuracy of the qualitative and quantitative model reaches 85.71%. Compared with the verification accuracy of 71.43% when inputting MACCS fingerprints into the DNN model, the accuracy of the qualitative and quantitative model is increased by 14.28%. Comparison Accuracy at a Glance Figure 9 shown.
PUM
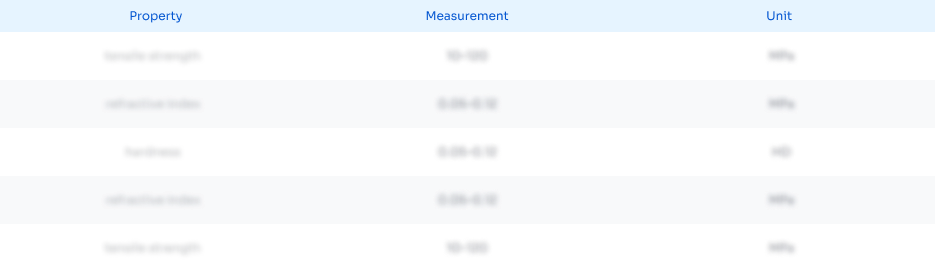
Abstract
Description
Claims
Application Information
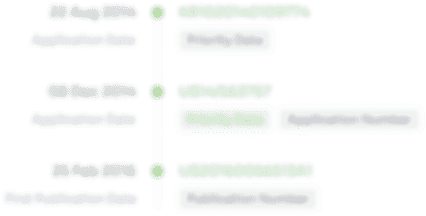
- R&D Engineer
- R&D Manager
- IP Professional
- Industry Leading Data Capabilities
- Powerful AI technology
- Patent DNA Extraction
Browse by: Latest US Patents, China's latest patents, Technical Efficacy Thesaurus, Application Domain, Technology Topic, Popular Technical Reports.
© 2024 PatSnap. All rights reserved.Legal|Privacy policy|Modern Slavery Act Transparency Statement|Sitemap|About US| Contact US: help@patsnap.com