QSM deep brain nucleus automatic segmentation method based on deep learning
A deep learning and automatic segmentation technology, applied in the field of neuroimaging, can solve the problems of high computational cost, not fully automatic, and time-consuming
- Summary
- Abstract
- Description
- Claims
- Application Information
AI Technical Summary
Problems solved by technology
Method used
Image
Examples
Embodiment Construction
[0033] An automatic segmentation method of QSM deep brain nuclei based on deep learning in the present invention is implemented based on a rapid segmentation tool for deep subcortical gray matter nuclei of QSM, named DeepQSMSeg. The segmentation subject of the present invention is a single-stage 3D encoder-decoder fully convolutional network (FCN). The network subject consists of an encoder (left part) and a decoder (right part). The encoder consists of an input module and four The feature extraction module is composed; the decoder is composed of four feature reconstruction modules and an output module, which is symmetrical to the encoder. We manually annotated 5 pairs of DGM structures of deep subcortical gray matter nuclei. All these target structures are very small, so the foreground and background voxel numbers are quite unbalanced. Therefore, we adopt the attention module, and use dice loss and focal loss to jointly supervise the training process. Combining experience f...
PUM
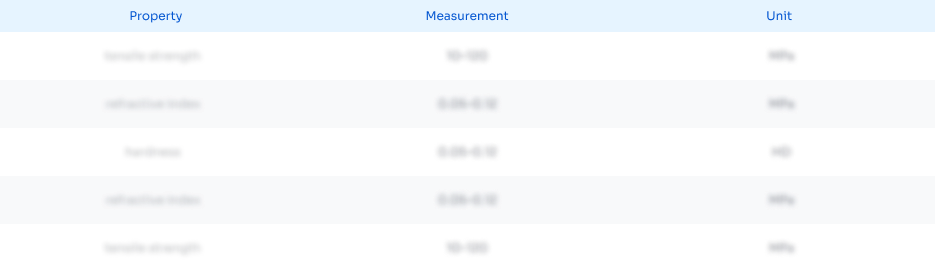
Abstract
Description
Claims
Application Information
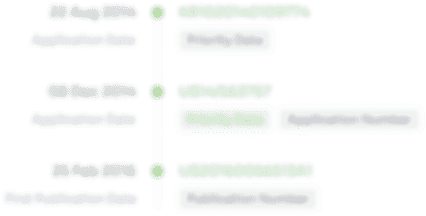
- R&D Engineer
- R&D Manager
- IP Professional
- Industry Leading Data Capabilities
- Powerful AI technology
- Patent DNA Extraction
Browse by: Latest US Patents, China's latest patents, Technical Efficacy Thesaurus, Application Domain, Technology Topic.
© 2024 PatSnap. All rights reserved.Legal|Privacy policy|Modern Slavery Act Transparency Statement|Sitemap