Real-time prediction method for aerodynamic performance parameters of automobile based on three-dimensional deep learning
A technology of aerodynamic performance and deep learning, applied in the field of aerodynamics and deep learning, can solve problems such as high-degree-of-freedom large-scale engineering problem dependence, computer performance requirements, and time-consuming problems, so as to speed up the design of aerodynamic layout and reduce R&D The effect of cycle and low cost
- Summary
- Abstract
- Description
- Claims
- Application Information
AI Technical Summary
Problems solved by technology
Method used
Image
Examples
Embodiment Construction
[0024] In order to more clearly illustrate the technical solutions in the embodiments of the present invention or the prior art, the following will briefly introduce the drawings that need to be used in the description of the embodiments or the prior art. Obviously, the accompanying drawings in the following description These are some embodiments of the present invention. Those skilled in the art can also obtain other drawings based on these drawings without creative work.
[0025] A real-time prediction method of automobile aerodynamic performance parameters based on three-dimensional deep learning proposed by the present invention, such as figure 1 As shown, it specifically includes the following steps:
[0026] S1. Obtain the 3D digital model and point cloud data of the car body, and process the data; the specific process is as follows: use T-Splines to construct the car shape surface model, then extract the surface control points and output the 3D coordinates of each point...
PUM
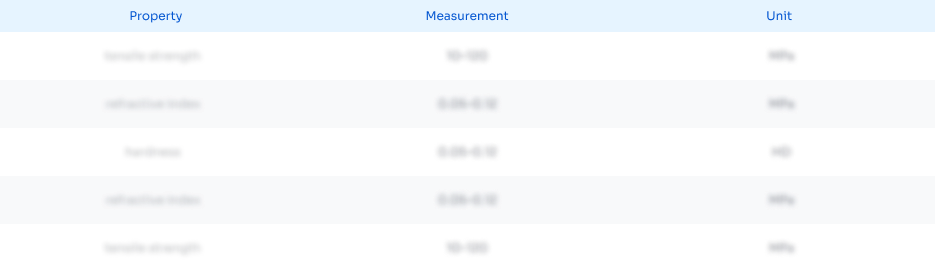
Abstract
Description
Claims
Application Information
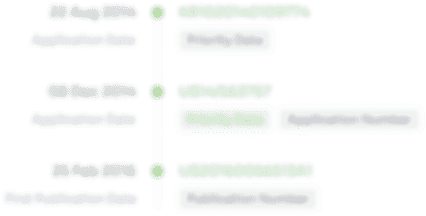
- R&D Engineer
- R&D Manager
- IP Professional
- Industry Leading Data Capabilities
- Powerful AI technology
- Patent DNA Extraction
Browse by: Latest US Patents, China's latest patents, Technical Efficacy Thesaurus, Application Domain, Technology Topic, Popular Technical Reports.
© 2024 PatSnap. All rights reserved.Legal|Privacy policy|Modern Slavery Act Transparency Statement|Sitemap|About US| Contact US: help@patsnap.com