Domain adaptive unsupervised image segmentation method based on generative adversarial and class feature distribution
A feature distribution and image segmentation technology, which is applied in image analysis, image enhancement, image data processing, etc., can solve the problems that class-level segmentation cannot be eliminated and overall information is ignored, so as to enhance multi-granularity semantic consistency, improve accuracy, Solve the effect of insufficient segmentation accuracy
- Summary
- Abstract
- Description
- Claims
- Application Information
AI Technical Summary
Problems solved by technology
Method used
Image
Examples
Embodiment 1
[0047] See attached figure 1 , the framework of the present invention is mainly two branches to process the images of two domains respectively: the image source domain image processed by branch a (the network processing flow is represented by a solid line in the figure) is obtained through the main semantic segmentation network to obtain the segmentation map, and the segmentation Figure 1 On the one hand, do cross-entropy loss with the real image, on the other hand, as part of the input training discriminator D; at the same time, the source domain image obtains the feature C of the category center through the auxiliary segmentation network s Indicates the input pseudo-label optimization module group to optimize the target domain pseudo-label. The target domain image processed by the other branch b (the dotted line in the figure indicates the network processing flow) is obtained through the main segmentation network to obtain the segmentation image, one way is input into the p...
PUM
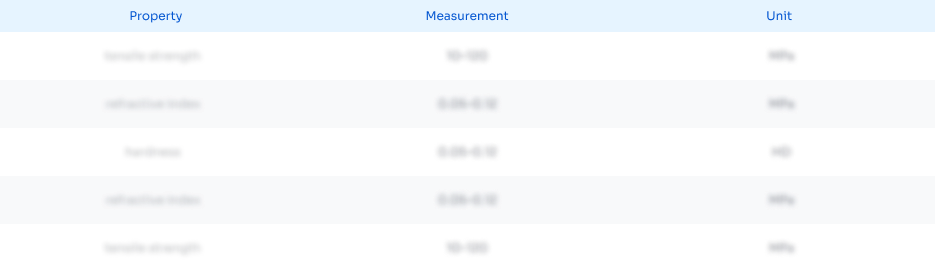
Abstract
Description
Claims
Application Information
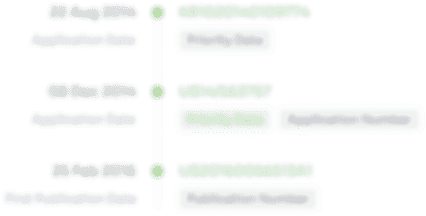
- R&D Engineer
- R&D Manager
- IP Professional
- Industry Leading Data Capabilities
- Powerful AI technology
- Patent DNA Extraction
Browse by: Latest US Patents, China's latest patents, Technical Efficacy Thesaurus, Application Domain, Technology Topic, Popular Technical Reports.
© 2024 PatSnap. All rights reserved.Legal|Privacy policy|Modern Slavery Act Transparency Statement|Sitemap|About US| Contact US: help@patsnap.com