A dynamic detection method for distribution transformer faults based on aoelm
A technology for distribution transformers and transformer faults is applied in the field of dynamic detection of low-voltage transformer faults in distribution networks based on active online extreme learning machines. Distributed drift characteristics and other problems, to achieve the effect of solving the active online dynamic update capability, reducing the fault false alarm rate, and ensuring the detection accuracy rate
- Summary
- Abstract
- Description
- Claims
- Application Information
AI Technical Summary
Problems solved by technology
Method used
Image
Examples
Embodiment Construction
[0041] The following will clearly and completely describe the technical solutions in the embodiments of the present invention with reference to the accompanying drawings in the embodiments of the present invention. Obviously, the described embodiments are only some, not all, embodiments of the present invention. Based on the embodiments of the present invention, all other embodiments obtained by persons of ordinary skill in the art without making creative efforts belong to the protection scope of the present invention.
[0042] The purpose of the present invention is to provide a dynamic detection method for distribution transformer faults based on AOELM to solve the problem that existing static detection methods for transformer faults do not have active online dynamic update capabilities and cannot adapt to the data distribution drift characteristics in the time-varying process problems, and further reduce the false positive rate of faults while ensuring the accuracy of fault ...
PUM
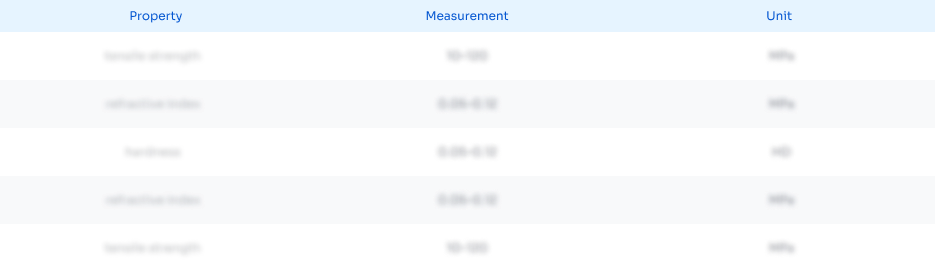
Abstract
Description
Claims
Application Information
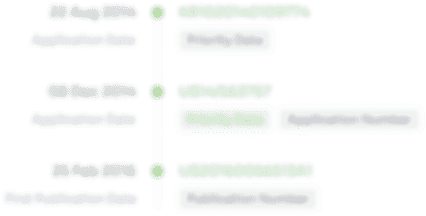
- R&D Engineer
- R&D Manager
- IP Professional
- Industry Leading Data Capabilities
- Powerful AI technology
- Patent DNA Extraction
Browse by: Latest US Patents, China's latest patents, Technical Efficacy Thesaurus, Application Domain, Technology Topic.
© 2024 PatSnap. All rights reserved.Legal|Privacy policy|Modern Slavery Act Transparency Statement|Sitemap