Non-uniform fog image defogging algorithm based on transmission attention mechanism
A kind of attention and non-uniform technology, applied in the field of image processing, can solve the problems of haze residue, non-uniform haze image and unsatisfactory defogging effect, etc.
- Summary
- Abstract
- Description
- Claims
- Application Information
AI Technical Summary
Problems solved by technology
Method used
Image
Examples
Embodiment 1
[0044] combine figure 1, a non-uniform fog image defogging algorithm based on a transfer attention mechanism, characterized in that it includes the following steps:
[0045] S1. Sparse and smooth atrous convolution feature extraction, constructing sparse blocks in the form of interleaved ordinary convolution and smooth atrous convolution, extracting feature information of different levels in the image;
[0046] S2. Non-uniform haze feature processing based on the transfer attention mechanism, integrating the attention mechanism into the dehazing network, learning the channel attention feature map and pixel attention feature map in different levels of feature maps, and giving each channel a different The weight value and make the network pay more attention to the dense fog area and high-frequency area, adaptively learn the feature map of the feature map under different weights, and then combine the channel attention feature map and the pixel attention feature map in the attenti...
Embodiment 2
[0050] The overall structure of the transfer attention dehazing network is as follows: figure 2 As shown, the main part of the network is inside the dotted line box. First, the feature information of the input image is initially extracted through three convolutional layers (Conv1~3). The step size of Conv3 is 2, which can reduce the size of the feature map and reduce the network performance. Computational complexity.
[0051] Then, five cascaded sparse blocks (S-1~S-5) are used to extract the features of different layers in the image, avoiding the use of down-sampling operations and reducing the loss of detail information.
[0052] Next, the gated fusion network is used to directly perform gated fusion on the extracted feature maps of the low, medium, and high levels, effectively aggregate image features at different levels, and obtain feature maps containing rich feature information.
[0053] Finally, the fused feature map is restored to the same size as the input fog map b...
Embodiment 3
[0056] Sparse Smooth Atrous Convolutional Feature Extraction.
[0057] The image classification network based on deep learning often expands the receptive field through multi-layer down-sampling operations in order to extract high-level semantic features in the image and reduce the amount of calculation while improving the overall performance of the network. However, the image dehazing network is a reconstruction process at the pixel level , a large number of downsampling operations are easy to lose image detail information, which brings great challenges to the reconstruction of haze-free images. Therefore, it is particularly important to preserve as much detail information as possible while expanding the image receptive field.
[0058] This application proposes a smooth atrous convolution with a sparse structure to achieve feature extraction, and uses gated fusion to fuse features of different levels.
[0059] The sparse mechanism can be effectively applied in the field of im...
PUM
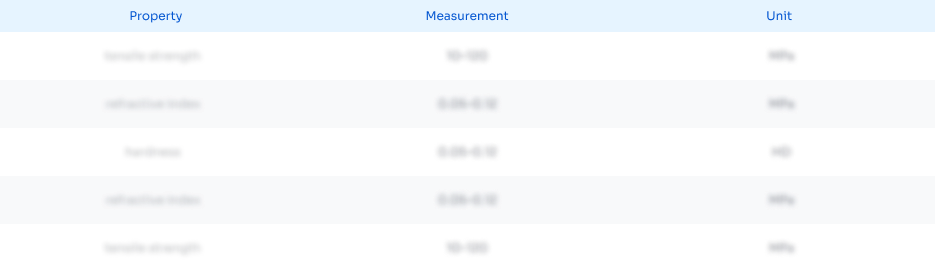
Abstract
Description
Claims
Application Information
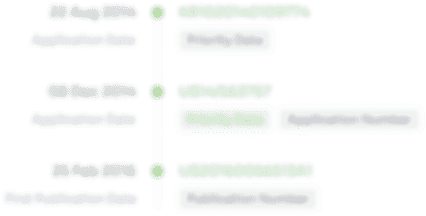
- R&D Engineer
- R&D Manager
- IP Professional
- Industry Leading Data Capabilities
- Powerful AI technology
- Patent DNA Extraction
Browse by: Latest US Patents, China's latest patents, Technical Efficacy Thesaurus, Application Domain, Technology Topic, Popular Technical Reports.
© 2024 PatSnap. All rights reserved.Legal|Privacy policy|Modern Slavery Act Transparency Statement|Sitemap|About US| Contact US: help@patsnap.com