Cross-domain pedestrian re-identification method and system based on multi-feature mixed learning
A technology of pedestrian re-identification and mixed learning, applied in neural learning methods, character and pattern recognition, instruments, etc., can solve the problem of less multi-feature mixed learning, improve self-adaptive ability, reduce inter-domain differences, and improve performance effect
- Summary
- Abstract
- Description
- Claims
- Application Information
AI Technical Summary
Problems solved by technology
Method used
Image
Examples
Embodiment 1
[0042] Embodiment 1 of the present invention provides a cross-domain pedestrian re-identification system based on multi-feature hybrid learning, which includes:
[0043] The extraction module is used to extract the pedestrian image to be recognized and the pedestrian global feature, pedestrian attribute feature and pedestrian attribute feature of the pedestrian image in the image base library gallery that are similar to the pedestrian identity in the pedestrian image to be recognized by using the re-identification model that has been jointly trained. Pedestrian local features; where the re-identification model is trained using the source domain with identity labels and attribute pseudo-labels and the target domain with identity pseudo-labels;
[0044] The identification module is used to fuse the pedestrian global features, pedestrian attribute features and pedestrian local features to be identified, and perform similarity matching and sorting with the features after the fusion o...
Embodiment 2
[0065] Embodiment 2 provides a cross-domain pedestrian re-identification method based on multi-feature hybrid learning, the method comprising:
[0066] Step S0, data collection. Cross-domain and cross-camera data collection, record the source domain data set as S, S domain has identity ID labels and attribute pseudo-labels, record the target domain data set as T, T domain only has attribute pseudo-labels, and divide each data set into a training set and the test set;
[0067] Step S1, preprocessing. Perform image preprocessing on consecutive screenshots of the video in the collected data set, such as scaling, cropping, averaging, normalization, etc., try to have multiple full-body photos of the same person;
[0068] Step S2, blended learning. Through the source domain learning of global and local features and the joint learning of attribute features, that is, joint training is performed on the source domain data set S and the target domain data set T at the same time.
[0...
Embodiment 3
[0083]Embodiment 3 of the present invention provides a cross-domain pedestrian re-identification method based on multi-feature hybrid learning. Taking a certain pedestrian recognition data set S as an example, it contains 6 different viewing angle camera data, and the training set contains 12936 images, a total of 751 People, with an average of 17.2 training data per person, the test set contains 19,732 images, a total of 750 people; taking a pedestrian recognition data set T as an example, it contains 8 camera data from different perspectives, and the training set contains 16,522 images, a total of 702 People, with an average of 23.5 training data per person, and the test set contains 17661 images.
[0084] The pedestrian re-identification method described in Embodiment 3 specifically includes the following steps:
[0085] Step S0, data collection. The above-mentioned pedestrian recognition data set S is recorded as the source domain data set S, and the S domain has identity...
PUM
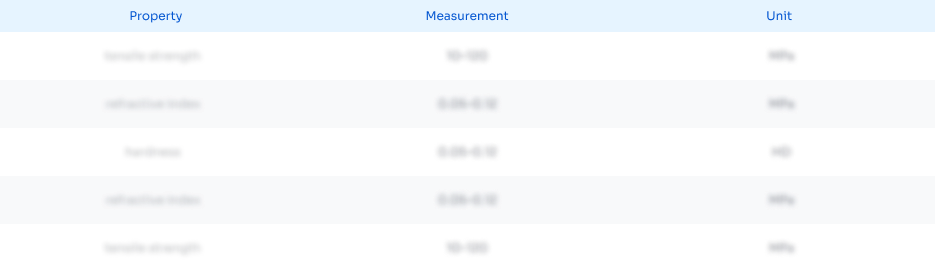
Abstract
Description
Claims
Application Information
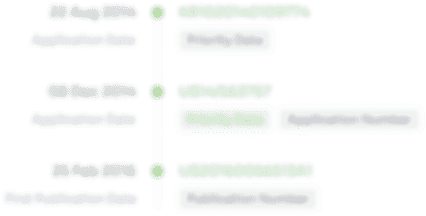
- R&D Engineer
- R&D Manager
- IP Professional
- Industry Leading Data Capabilities
- Powerful AI technology
- Patent DNA Extraction
Browse by: Latest US Patents, China's latest patents, Technical Efficacy Thesaurus, Application Domain, Technology Topic, Popular Technical Reports.
© 2024 PatSnap. All rights reserved.Legal|Privacy policy|Modern Slavery Act Transparency Statement|Sitemap|About US| Contact US: help@patsnap.com