A fully convolutional network-based oil spill detection method on the sea surface and its system and application
A fully convolutional network, sea surface oil spill technology, applied in the field of sea surface oil spill detection, can solve the problems of difficult segmentation of oil spill and sea water background, inability to take into account detection accuracy and speed, low segmentation accuracy, etc., to reduce memory and Complex calculation, solving scale change problem, the effect of less calculation
- Summary
- Abstract
- Description
- Claims
- Application Information
AI Technical Summary
Problems solved by technology
Method used
Image
Examples
Embodiment 1
[0100] like figure 1 As shown, a method flow chart of a fully convolutional network-based sea surface oil spill detection method is disclosed, and the specific operations are as follows:
[0101] 1) First, obtain an image of oil spill on the sea surface, and perform a preprocessing operation on the oil spill image to obtain an image data set. The oil spill image is input into a fully convolutional network to perform binary segmentation of the oil film area and the background image, and then the spectrogram of the oil film area is extracted.
[0102] 2) combining the image data set with the spectrogram of the oil film as a training sample set; labeling the training sample set to obtain label information of the training sample set;
[0103] then input the label information of the training sample set into the pre-built initial sea surface oil spill detection network;
[0104] 3) The parameters of the initial sea surface oil spill detection network are optimized by the back prop...
Embodiment 2
[0108] like figure 2 As shown, it is a flow chart of a sea surface oil spill detection method based on a fully convolutional network:
[0109] Step S01: simulate a sea surface oil spill site in the laboratory and take an oil spill image as a data training set, perform preprocessing on the oil spill image and segment it to obtain an oil film area, and obtain a spectrogram obtained by two-dimensional Fourier transform and annotated images together as a training sample set.
[0110] The preprocessing operation mainly includes: (1) The adaptive histogram equalization method for limiting contrast solves the problem of unsatisfactory contrast while suppressing the enhancement of noise. (2) The high-frequency enhancement filtering method sharpens and enhances the edge of the image, and stretches the grayscale distribution of the image to a certain extent. The main purpose is to make the oil spill image clearer and enhance the contrast of seawater and oil film to improve segmentati...
Embodiment 3
[0118] like Figure 4 As shown, this embodiment provides a sea surface oil spill detection system based on a fully convolutional network, which is an end-to-end one-stage anchor-free detection network, including a backbone network, a Transformer module, a Transformed feature pyramid, and a head network.
[0119] The detection network is based on full convolution and is used to predict the class information and regression information of each pixel in the image.
[0120] The backbone network adopts ResNet-50 network, which is used to extract and save the feature information of oil spill images.
[0121] The Transformer module mainly includes single-scale transformation, cross-scale transformation and Gateblock, and increasing the global information of features is beneficial to the detection of large-area oil films. ;
[0122] The Transformed feature pyramid solves the problem of scale change, and at the same time obtains multi-scale context information to establish the connect...
PUM
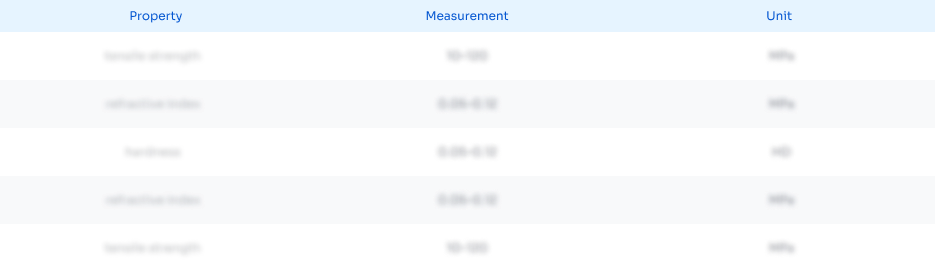
Abstract
Description
Claims
Application Information
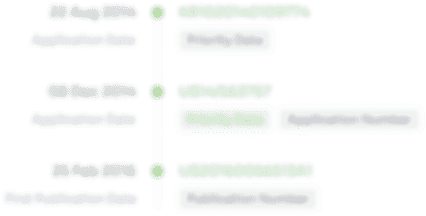
- R&D Engineer
- R&D Manager
- IP Professional
- Industry Leading Data Capabilities
- Powerful AI technology
- Patent DNA Extraction
Browse by: Latest US Patents, China's latest patents, Technical Efficacy Thesaurus, Application Domain, Technology Topic, Popular Technical Reports.
© 2024 PatSnap. All rights reserved.Legal|Privacy policy|Modern Slavery Act Transparency Statement|Sitemap|About US| Contact US: help@patsnap.com