Time series prediction method combined with multiple models
A technology of time series and forecasting methods, applied in forecasting, neural learning methods, biological neural network models, etc., can solve the problem of not being able to accurately capture and fit time series features, not being able to really improve the accuracy of forecasting, and not being able to mine time series Problems such as trends and seasonal laws can achieve high-precision results
- Summary
- Abstract
- Description
- Claims
- Application Information
AI Technical Summary
Problems solved by technology
Method used
Image
Examples
Embodiment Construction
[0046] The present invention will be described in further detail below in conjunction with the accompanying drawings and specific embodiments. It should be understood that the specific embodiments described here are only used to explain the present invention, not to limit the present invention.
[0047] The main problem of time series forecasting is that it is impossible to extract the salient features of time series for forecasting. In order to achieve a more accurate prediction effect, such as figure 1 and figure 2 As shown, the present invention provides a time series forecasting method that combines multiple models, and uses a time series decomposition algorithm to decompose the time series into three time series: long-term trend, seasonal fluctuation, and irregular fluctuation. In this way, a time series with long-term trend characteristics, a time series with seasonal fluctuation characteristics, and a time series with irregular fluctuations will be obtained. By usin...
PUM
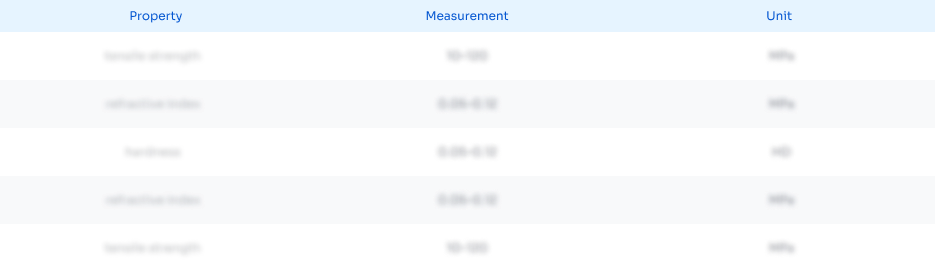
Abstract
Description
Claims
Application Information
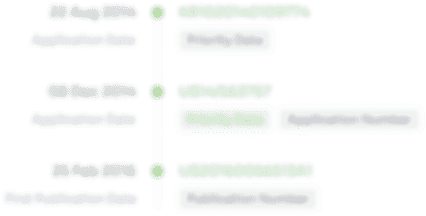
- R&D Engineer
- R&D Manager
- IP Professional
- Industry Leading Data Capabilities
- Powerful AI technology
- Patent DNA Extraction
Browse by: Latest US Patents, China's latest patents, Technical Efficacy Thesaurus, Application Domain, Technology Topic, Popular Technical Reports.
© 2024 PatSnap. All rights reserved.Legal|Privacy policy|Modern Slavery Act Transparency Statement|Sitemap|About US| Contact US: help@patsnap.com