A Hyperspectral Remote Sensing Image Classification Method Based on Dual Attention Mechanism
A hyperspectral remote sensing and attention technology, applied in the field of hyperspectral remote sensing images, can solve the problems of unsatisfactory, limited classification accuracy of training samples of hyperspectral remote sensing images, etc., to achieve good performance and reduce data redundancy.
- Summary
- Abstract
- Description
- Claims
- Application Information
AI Technical Summary
Problems solved by technology
Method used
Image
Examples
Embodiment 1
[0180] Embodiment 1: In order to verify the impact of different parameter settings on the classification accuracy in the method proposed by the present invention (HDDA), the input sample window size w, the learning rate lr, and the Dropout parameters were mainly analyzed. In this embodiment, 5%, 1%, and 1% of the training samples are randomly selected from each type of ground objects in the IP, UP, and SA data sets to train the model, and the rest are used as test samples. In addition, the dimensionality reduction dimension d of the present invention is set to 10, and the HDDA network model is trained by using the MSE loss function and the Adam optimization algorithm. The batch size is uniformly 64, and the iteration is 200 times. Figure 5 (a) is the influence of the method HDDA of the present invention on the classification accuracy under different window sizes w. If the w value is small, the receptive field of the convolution kernel feature extraction will be insufficient, ...
Embodiment 2
[0184] Embodiment 2: In order to further verify the effectiveness of the algorithm of the present invention, this embodiment adopts three kinds of data sets of IP, UP, and SA for verification, and randomly selects 5%, 1%, and 1% from each type of ground object as training samples set, and the rest as the test sample set. And use REF-SVM, 3D-CNN, HybridSN, SSRN and R-HybridSN five hyperspectral image classification methods as the comparative experimental objects, the classification results take the mean of ten experimental results, and record the standard deviation, so as to verify the HDDA method classification performance.
[0185] Table 3. Classification accuracy of different classification methods on the IP dataset.
[0186]
[0187] right figure 2The shown IP hyperspectral image is classified, and the HDDA classification method proposed by the present invention uses a 5-layer stacked autoencoder structure to reduce the dimensionality of the hyperspectral remote sensi...
Embodiment 3
[0194] Embodiment 3: In order to further verify the classification performance of the HDDA classification method of the present invention under limited training samples, this embodiment sets different training sample ratios on the IP, UP, and SA data sets respectively, and sets respectively on the IP data set 2%, 4%, 6%, 8%, 10% five sets of training sample proportions, set 0.2%, 0.4%, 0.6%, 0.8% four sets of training sample proportions on the UP and SA data sets, by further reducing the training samples , so as to compare the overall classification accuracy of the classification method of the present invention and the comparative classification method.
[0195] Figure 10 Influence of HDDA on hyperspectral image classification under different sample ratios for the method described in the present invention. from Figure 10 It can be seen that the HDDA classification method proposed by the present invention shows the best classification accuracy in OA and OA under different p...
PUM
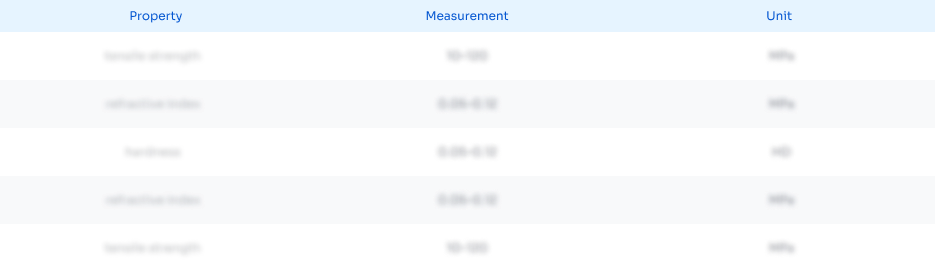
Abstract
Description
Claims
Application Information
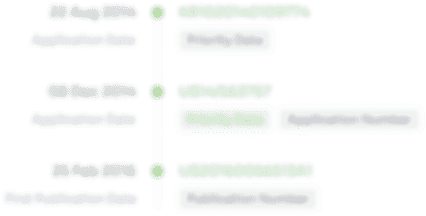
- Generate Ideas
- Intellectual Property
- Life Sciences
- Materials
- Tech Scout
- Unparalleled Data Quality
- Higher Quality Content
- 60% Fewer Hallucinations
Browse by: Latest US Patents, China's latest patents, Technical Efficacy Thesaurus, Application Domain, Technology Topic, Popular Technical Reports.
© 2025 PatSnap. All rights reserved.Legal|Privacy policy|Modern Slavery Act Transparency Statement|Sitemap|About US| Contact US: help@patsnap.com