Hyperspectral image joint classification method based on multi-feature learning and superpixel kernel sparse representation
A kernel sparse representation, hyperspectral image technology, applied in the field of practical engineering and hyperspectral data processing, to achieve the effect of improving classification accuracy, improving classification accuracy and high classification accuracy
Active Publication Date: 2020-03-06
NANJING UNIV OF AERONAUTICS & ASTRONAUTICS
View PDF2 Cites 4 Cited by
- Summary
- Abstract
- Description
- Claims
- Application Information
AI Technical Summary
Problems solved by technology
Existing multi-feature-based classification methods still have obvious problems, such as how to fully consider the similarity and diversity among different features
Method used
the structure of the environmentally friendly knitted fabric provided by the present invention; figure 2 Flow chart of the yarn wrapping machine for environmentally friendly knitted fabrics and storage devices; image 3 Is the parameter map of the yarn covering machine
View moreImage
Smart Image Click on the blue labels to locate them in the text.
Smart ImageViewing Examples
Examples
Experimental program
Comparison scheme
Effect test
Embodiment 1
[0092] In order to better reflect the advantages of the hyperspectral image classification method based on multi-feature learning and superpixel kernel sparse representation of the present invention, the classification method described in the present invention and the existing classic classification methods SVM and SRC will be combined with a specific example below. , JSRC, SAJSRC, SPJSRC, MFASRC, SSFL-KSRC and SPFL-KSRC for comparison.
the structure of the environmentally friendly knitted fabric provided by the present invention; figure 2 Flow chart of the yarn wrapping machine for environmentally friendly knitted fabrics and storage devices; image 3 Is the parameter map of the yarn covering machine
Login to View More PUM
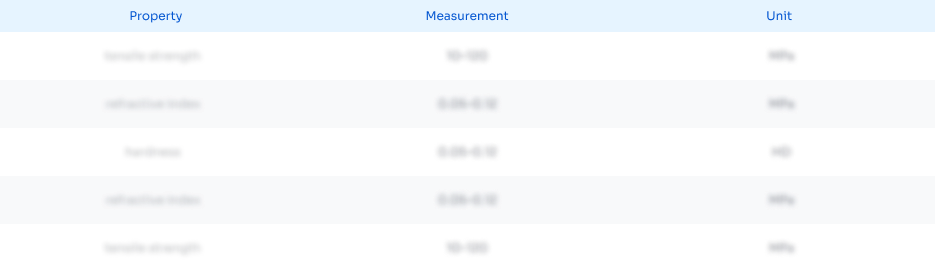
Abstract
The invention discloses a hyperspectral image joint classification method based on multi-feature learning and superpixel kernel sparse representation. used for improving the classification precision of hyperspectral images. According to the method, spatial features and local and global super-pixel features are extracted firstly, and spatial information is fully utilized to improve the classification precision; then, a kernel sparse representation classification method based on multiple features is provided, and the classification problem that a high-dimensional feature space is linearly inseparable is effectively solved; moreover, the similarity and diversity among multiple features are fully utilized, a multi-kernel learning method is used for constructing a basic kernel and optimizing the coefficient of the basic kernel, and a composite kernel is designed to replace a single kernel in a kernel sparse representation method, so that the classification precision is further improved. Compared with similar classic hyperspectral image classification methods, the classification method is higher in classification precision and can be applied to the fields of hyperspectral classification,aerospace remote sensing and the like.
Description
technical field [0001] The invention relates to a hyperspectral image joint classification method based on multi-feature learning and superpixel kernel sparse representation, which belongs to the technical field of hyperspectral data processing, is used to effectively improve the classification accuracy in the case of small samples, and can be applied to aerospace remote sensing detection and other practical applications engineering field. Background technique [0002] Hyperspectral image classification technology is a hot research issue in the field of remote sensing, and its goal is to classify each spectral pixel into a specific category. In recent years, many classification methods have been proposed, including MLR, SVM and neural network. However, these methods only exploit the spectral information of hyperspectral data and ignore the spatial information, thus leading to noisy classification results. In order to overcome this problem, many methods that try to combine ...
Claims
the structure of the environmentally friendly knitted fabric provided by the present invention; figure 2 Flow chart of the yarn wrapping machine for environmentally friendly knitted fabrics and storage devices; image 3 Is the parameter map of the yarn covering machine
Login to View More Application Information
Patent Timeline
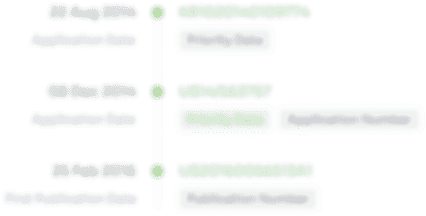
Patent Type & Authority Applications(China)
IPC IPC(8): G06K9/00G06K9/62
CPCG06V20/13G06F18/2135G06F18/24Y02A40/10
Inventor 李丹孔繁锵
Owner NANJING UNIV OF AERONAUTICS & ASTRONAUTICS
Features
- Generate Ideas
- Intellectual Property
- Life Sciences
- Materials
- Tech Scout
Why Patsnap Eureka
- Unparalleled Data Quality
- Higher Quality Content
- 60% Fewer Hallucinations
Social media
Patsnap Eureka Blog
Learn More Browse by: Latest US Patents, China's latest patents, Technical Efficacy Thesaurus, Application Domain, Technology Topic, Popular Technical Reports.
© 2025 PatSnap. All rights reserved.Legal|Privacy policy|Modern Slavery Act Transparency Statement|Sitemap|About US| Contact US: help@patsnap.com