Penicillin fermentation process fault prediction method based on Attention-LSTM of multivariate time series
A multivariate time series, penicillin fermentation technology, applied in neural learning methods, complex mathematical operations, biological neural network models, etc., can solve problems such as insufficient long-term memory ability, gradient disappearance, gradient explosion, etc., to improve the accuracy and accuracy of system prediction performance and accurate fault prediction
- Summary
- Abstract
- Description
- Claims
- Application Information
AI Technical Summary
Problems solved by technology
Method used
Image
Examples
Embodiment
[0095] 1) Use the penicillin simulation platform Pensim to simulate and generate 20 batches of normal fermentation process data with a sampling time of 400h and a sampling interval of 1h, as the control limit for predicting whether a failure occurs and SPE cl ; Collect 20 batches of normal fermentation process data with a sampling time of 400 h and a sampling interval of 1 h and 1 batch of fault data with a slope of 5% stirring power introduced at a sampling time of 320 h as sample data, of which 20 batches of normal fermentation process data are used as Training set, 1 batch of fault data as a test set;
[0096] 3) respectively for and SPE train Do correlation analysis with the on-line measurable variable in the penicillin fermentation process and adopt Pearson (Pearson) correlation analysis, analysis result is as shown in table 1:
[0097] Table 1
[0098]
[0099] The present invention selects the on-line measurable process variables with P0.2 as significantly rele...
PUM
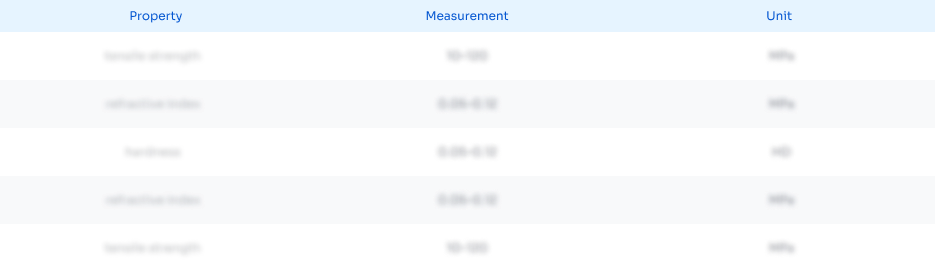
Abstract
Description
Claims
Application Information
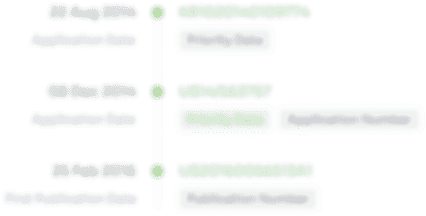
- R&D Engineer
- R&D Manager
- IP Professional
- Industry Leading Data Capabilities
- Powerful AI technology
- Patent DNA Extraction
Browse by: Latest US Patents, China's latest patents, Technical Efficacy Thesaurus, Application Domain, Technology Topic, Popular Technical Reports.
© 2024 PatSnap. All rights reserved.Legal|Privacy policy|Modern Slavery Act Transparency Statement|Sitemap|About US| Contact US: help@patsnap.com