Multi-objective battery pack structure optimization method based on neural network
A multi-objective optimization and neural network technology, applied in the field of battery systems, can solve problems such as unreasonable battery pack structure settings, consuming a lot of time, and consuming a lot of time
- Summary
- Abstract
- Description
- Claims
- Application Information
AI Technical Summary
Problems solved by technology
Method used
Image
Examples
Embodiment
[0031] Embodiment: a kind of multi-objective optimization battery pack structure method based on neural network, such as figure 1 shown, including the following steps:
[0032] S1: Convert the 3D model of the battery pack to a 2D model and perform simulation in COMSOL to generate multiple sets of structural parameters that affect the performance of the battery pack; since the calculation of the 3D battery pack takes several hours to obtain the numerical results, and the 2D battery pack The calculation only takes a few minutes, so the 2D battery pack can be used instead of the 3D battery pack for simulation. In order to simplify the structure of the battery pack, the 3D model of the battery pack is axisymmetric, ignoring the small details in the battery pack. The air-cooled 3D battery Package diagram such as figure 2 shown. The lower left side of the battery pack is the air inlet for cooling air, while the upper right side is the air outlet for the air. The air enters the ba...
PUM
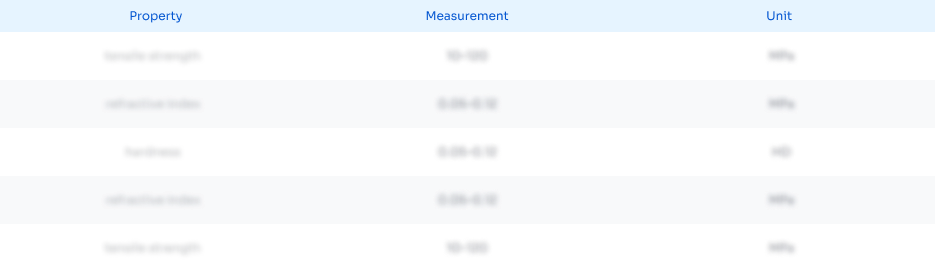
Abstract
Description
Claims
Application Information
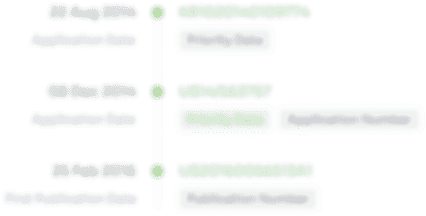
- R&D Engineer
- R&D Manager
- IP Professional
- Industry Leading Data Capabilities
- Powerful AI technology
- Patent DNA Extraction
Browse by: Latest US Patents, China's latest patents, Technical Efficacy Thesaurus, Application Domain, Technology Topic, Popular Technical Reports.
© 2024 PatSnap. All rights reserved.Legal|Privacy policy|Modern Slavery Act Transparency Statement|Sitemap|About US| Contact US: help@patsnap.com