Unsupervised medical image segmentation method based on adversarial network
A medical image, unsupervised technique used in healthcare informatics to solve problems such as unsupervised, low performance and complex steps
- Summary
- Abstract
- Description
- Claims
- Application Information
AI Technical Summary
Problems solved by technology
Method used
Image
Examples
Embodiment Construction
[0060] In combination with the content of the present invention, the following embodiments are provided for fetal head segmentation in ultrasonic images, optic disc segmentation in fundus images, lung segmentation in X-ray images, and liver segmentation in abdominal CT images. In this embodiment, the CPU is Intel(R) Core(TM) i7-6850K 3.60GHz, GPU is Nvidia GTX1080Ti, memory is 32.0GB, and the programming language is Python.
[0061] figure 1 Part (a) shows unpaired images and auxiliary masks, (b) shows an improved cycle-consistency adversarial network for unsupervised learning, (c) shows binary masks, and (d) shows parts of The process of exploiting binary mask learning is shown.
[0062] Step 1. Obtain the auxiliary mask
[0063] In the case of fetal head segmentation in ultrasound images and optic disc segmentation in fundus images, a set of random ellipses is generated as an auxiliary mask because both the fetal head and the optic disc are shaped like ellipses. For diffe...
PUM
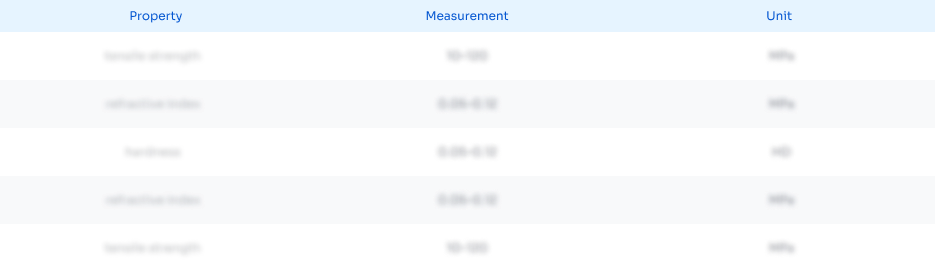
Abstract
Description
Claims
Application Information
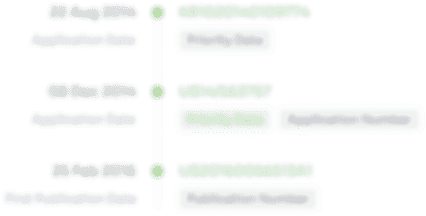
- R&D Engineer
- R&D Manager
- IP Professional
- Industry Leading Data Capabilities
- Powerful AI technology
- Patent DNA Extraction
Browse by: Latest US Patents, China's latest patents, Technical Efficacy Thesaurus, Application Domain, Technology Topic, Popular Technical Reports.
© 2024 PatSnap. All rights reserved.Legal|Privacy policy|Modern Slavery Act Transparency Statement|Sitemap|About US| Contact US: help@patsnap.com