CNN-LSTM fan fault prediction method and CNN-LSTM fan fault prediction system based on attention mechanism
A technology of fault prediction and attention, applied in neural learning methods, computer components, instruments, etc., can solve problems such as inability to mine time series features between data well, poor performance in dealing with time series problems, and poor generalization ability
- Summary
- Abstract
- Description
- Claims
- Application Information
AI Technical Summary
Problems solved by technology
Method used
Image
Examples
Embodiment 1
[0091] Example 1: A CNN-LSTM Fan Fault Prediction Method Based on Attention Mechanism
[0092] Such as image 3 As shown, Embodiment 1 of the present invention provides a CNN-LSTM fan failure prediction method based on attention mechanism, comprising the following steps:
[0093] Step 1. Collect fan timing data from the SCADA (Supervisory Control And Data Acquisition) system, including: its own performance data and external environment data, where its own performance data includes pitch angle, pitch speed, generator Current, power generation, etc.; external environment data include wind speed, temperature, humidity, etc.
[0094] It can be understood that those skilled in the art can arbitrarily configure the selected fan real-time data type, a preferred but non-limiting implementation is, in the embodiment of the present invention, in order to predict the icing failure of the fan, from the SCADA system The collected 370,000 pieces of data focus on wind speed, generator spee...
Embodiment 2
[0182] Example 2: CNN-LSTM Fan Fault Prediction System Based on Attention Mechanism
[0183] Such as figure 1 As shown, embodiment 2 of the present invention provides a kind of CNN-LSTM fan failure prediction system based on attention mechanism, including: input module, CNN neural network module, LSTM neural network module, attention mechanism module (Attention part) and output module.
[0184] Among them, the input module is used to input the data set filtered by the features of the random forest algorithm.
[0185] The CNN neural network module is used to compress the original features of the input data step by step, and finally obtain a high-level feature representation. The CNN neural network module includes:
[0186] The first part: the convolution layer, which consists of several convolution kernels. The weight of each convolution kernel is obtained through data-driven learning. These convolution kernels compress the original features of the input data step by step, a...
PUM
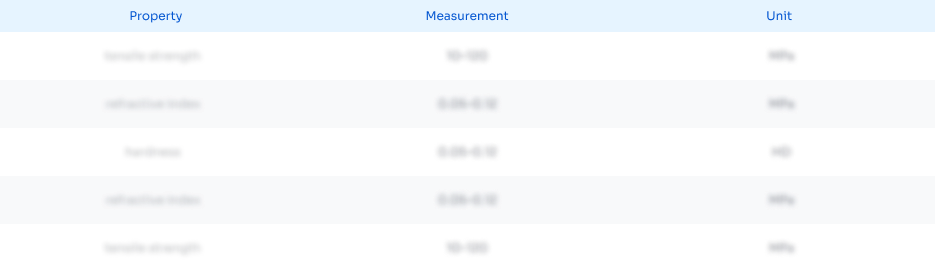
Abstract
Description
Claims
Application Information
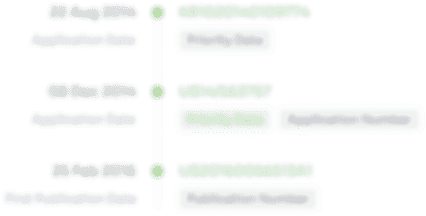
- R&D Engineer
- R&D Manager
- IP Professional
- Industry Leading Data Capabilities
- Powerful AI technology
- Patent DNA Extraction
Browse by: Latest US Patents, China's latest patents, Technical Efficacy Thesaurus, Application Domain, Technology Topic, Popular Technical Reports.
© 2024 PatSnap. All rights reserved.Legal|Privacy policy|Modern Slavery Act Transparency Statement|Sitemap|About US| Contact US: help@patsnap.com