Rolling bearing fault diagnosis method based on multi-feature extraction and WOA-ELM
A fault diagnosis, rolling bearing technology, applied in character and pattern recognition, instruments, calculation models, etc., can solve problems such as difficulty in reflecting the overall picture and local characteristics of time-frequency two domains, insensitivity to early faults of rolling bearings, and redundant characteristics, etc. The effect of improving the fault diagnosis rate
- Summary
- Abstract
- Description
- Claims
- Application Information
AI Technical Summary
Problems solved by technology
Method used
Image
Examples
Embodiment Construction
[0033] The present invention is described in detail below.
[0034] A Rolling Bearing Fault Diagnosis Method Based on Multi-Feature Extraction and WOA-ELM
[0035] Step 1, using the official bearing data of Casey Western Reserve University as the original vibration data;
[0036] Step 2, perform time domain analysis, spectrum analysis and wavelet packet decomposition on the original data, and extract time domain, frequency domain and time-frequency domain features;
[0037] Step 3, normalize the mixed domain feature set obtained in step 2;
[0038] Step 4, using the manifold learning LPP algorithm to perform dimension reduction on the normalized high-dimensional feature set to obtain a low-dimensional feature sample set;
[0039] Step five, use the WOA algorithm to optimize the parameters of the ELM network, and input the low-dimensional feature data to the WOA-ELM model for fault diagnosis.
[0040] The specific content of step two is: the time-domain feature is a dimensio...
PUM
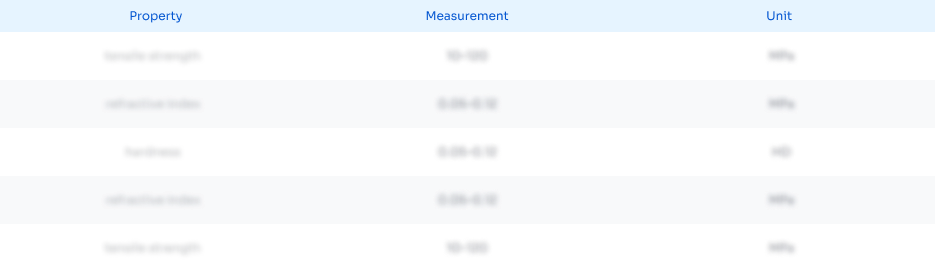
Abstract
Description
Claims
Application Information
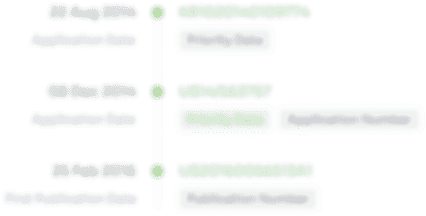
- R&D Engineer
- R&D Manager
- IP Professional
- Industry Leading Data Capabilities
- Powerful AI technology
- Patent DNA Extraction
Browse by: Latest US Patents, China's latest patents, Technical Efficacy Thesaurus, Application Domain, Technology Topic, Popular Technical Reports.
© 2024 PatSnap. All rights reserved.Legal|Privacy policy|Modern Slavery Act Transparency Statement|Sitemap|About US| Contact US: help@patsnap.com