Enteroscope withdrawal quality intelligent monitoring system and method based on deep neural network
A technology of deep neural network and intelligent monitoring system, which is applied in the direction of biological neural network model, neural architecture, image data processing, etc., and can solve the problems of colorectal endoscopy chaos and disorder
- Summary
- Abstract
- Description
- Claims
- Application Information
AI Technical Summary
Problems solved by technology
Method used
Image
Examples
Embodiment 1
[0040] This embodiment proposes a deep neural network-based intelligent monitoring system for the quality of colonoscope retraction. The schematic diagram of the system architecture is shown in figure 1 , where the system includes:
[0041]Background video processing and calling end, used to split the obtained video stream into image frames, and call the quality monitoring model;
[0042] The quality monitoring model end is used to run the target area detection model and classification model based on the deep neural network; the details include the following:
[0043] Invoke the target area detection model based on the deep neural network at startup, detect the target area in the frame image, and segment the detected target area from the corresponding image frame, and use the segmented target area for effective field of view Determine the validity, record the images of the effective field of view and the total number of images, and return the ratio of the number of images of ...
Embodiment 2
[0063] On the basis of Example 1, this embodiment proposes a method for intelligently monitoring the quality of colonoscope withdrawal based on deep neural networks, the flow chart of which is shown in Figure 5 , wherein the method includes the following steps:
[0064] S1. Acquire the video stream generated during colonoscopy.
[0065] S2. Split the acquired video stream into image frames.
[0066] S3. Detect the target area in each frame of image, and segment the detected target area from the corresponding image frame. Wherein, the frame image may include a target area part, a state information part and a control information part.
[0067] S4. Determine the validity of the field of view for the segmented target area, and record the images of the effective field of view and the total number of images.
[0068] S5. The ratio of the number of images in the effective field of view to the total number of images is returned to the video stream display end as the effective rate...
PUM
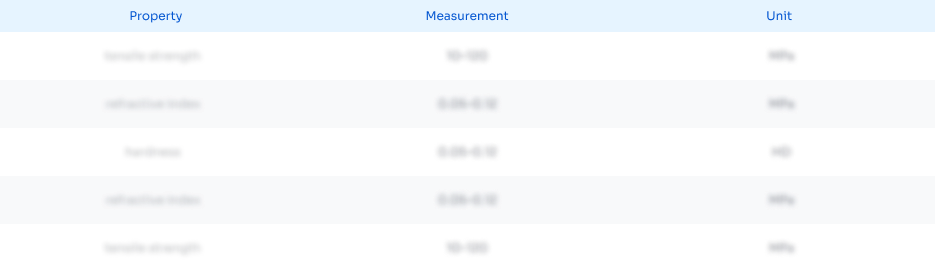
Abstract
Description
Claims
Application Information
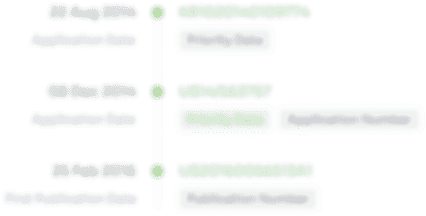
- R&D Engineer
- R&D Manager
- IP Professional
- Industry Leading Data Capabilities
- Powerful AI technology
- Patent DNA Extraction
Browse by: Latest US Patents, China's latest patents, Technical Efficacy Thesaurus, Application Domain, Technology Topic, Popular Technical Reports.
© 2024 PatSnap. All rights reserved.Legal|Privacy policy|Modern Slavery Act Transparency Statement|Sitemap|About US| Contact US: help@patsnap.com