Tunnel settlement time sequence prediction method based on CEEMDAN-BiLSTM
A technology of tunnel settlement and time series, applied in prediction, neural learning methods, biological neural network models, etc., can solve problems such as low prediction accuracy
- Summary
- Abstract
- Description
- Claims
- Application Information
AI Technical Summary
Problems solved by technology
Method used
Image
Examples
Embodiment Construction
[0025] The preferred embodiments of the present invention are described in detail below in conjunction with the accompanying drawings, but the present invention is not limited to these embodiments. The present invention covers any alternatives, modifications, equivalent methods and schemes made within the spirit and scope of the present invention.
[0026] In order to provide the public with a thorough understanding of the present invention, specific details are set forth in the following preferred embodiments of the present invention, but those skilled in the art can fully understand the present invention without the description of these details.
[0027] The empirical mode decomposition EMD algorithm decomposes the original sequence into multiple intrinsic mode functions with local time-varying characteristics, and predicts the characteristics of each function to form the final result. This method has excellent time-frequency aggregation, so it is very useful for the predicti...
PUM
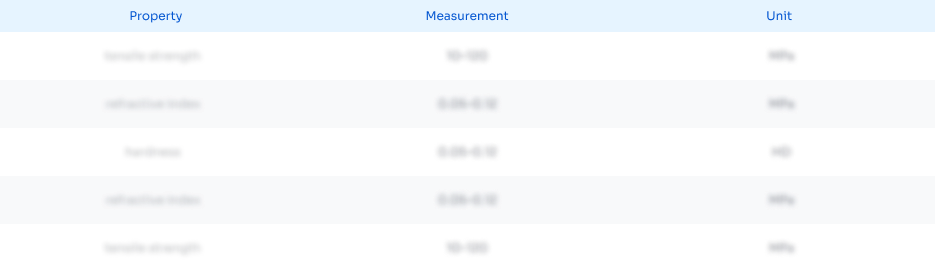
Abstract
Description
Claims
Application Information
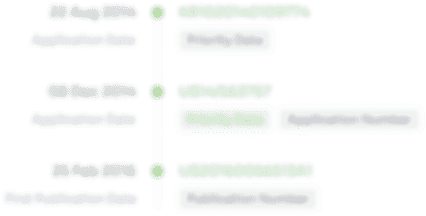
- R&D Engineer
- R&D Manager
- IP Professional
- Industry Leading Data Capabilities
- Powerful AI technology
- Patent DNA Extraction
Browse by: Latest US Patents, China's latest patents, Technical Efficacy Thesaurus, Application Domain, Technology Topic, Popular Technical Reports.
© 2024 PatSnap. All rights reserved.Legal|Privacy policy|Modern Slavery Act Transparency Statement|Sitemap|About US| Contact US: help@patsnap.com