Deep student performance prediction method based on multi-layer LSTM
A prediction method and student technology, applied in the research field of natural language processing sentiment analysis, can solve problems such as poor prediction effect and incomplete data utilization, and achieve the effect of improving prediction effect
- Summary
- Abstract
- Description
- Claims
- Application Information
AI Technical Summary
Problems solved by technology
Method used
Image
Examples
Embodiment
[0036] Such as figure 1As shown, a deep student performance prediction method based on multi-layer LSTM mainly includes three modules: data preprocessing, establishment of multi-layer LSTM structure model, and establishment of Softmax layer. The main processing processes are covered in the main in the module architecture.
[0037] Each part will be described in detail below.
[0038] S1. Data preprocessing
[0039] Preprocess the given student demographic data, such as gender, age range, and residential area, and perform One-Hot coding on it; organize the given student clickstream data by category on a weekly basis. The process framework of data preprocessing is as follows: figure 2 shown.
[0040] S1.1 Divide the data
[0041] Through a certain method, the demographics of the students are divided into sequence data and category data, and the click stream data of the students are sorted by the category of the click data on a weekly basis. The demographic data includes s...
PUM
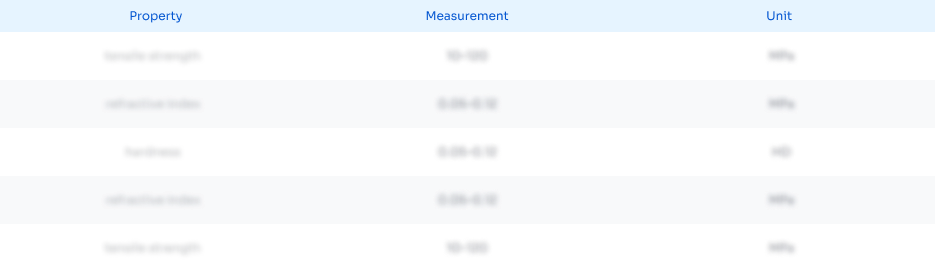
Abstract
Description
Claims
Application Information
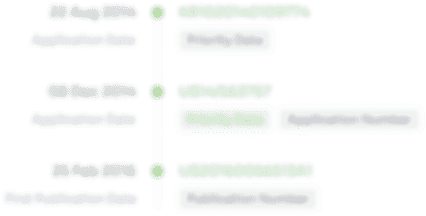
- R&D Engineer
- R&D Manager
- IP Professional
- Industry Leading Data Capabilities
- Powerful AI technology
- Patent DNA Extraction
Browse by: Latest US Patents, China's latest patents, Technical Efficacy Thesaurus, Application Domain, Technology Topic, Popular Technical Reports.
© 2024 PatSnap. All rights reserved.Legal|Privacy policy|Modern Slavery Act Transparency Statement|Sitemap|About US| Contact US: help@patsnap.com