Lateral chest radiography bone suppression method based on deep convolutional neural network
A convolutional neural network and neural network technology, which is applied in the field of lateral chest radiograph bone suppression based on deep convolutional neural network, can solve the problems of difficulty in decomposing lateral chest radiographs, overlapping and interlaced bones, and low contrast. Lung disease missed detection rate, improved accuracy, and the effect of avoiding occlusion
- Summary
- Abstract
- Description
- Claims
- Application Information
AI Technical Summary
Problems solved by technology
Method used
Image
Examples
Embodiment 1
[0050] A bone suppression method for lateral chest radiographs based on deep convolutional neural networks, such as Figures 1 to 5 As shown, the steps involved are:
[0051] Step 1, performing normalization processing on the gray space of the original lateral chest radiograph image to obtain a normalized processing image;
[0052] Perform differential operations on the horizontal and vertical directions of the original lateral chest image to obtain a lateral chest gradient image, and then normalize the gray-scale space of the lateral chest gradient image to obtain a normalized processed gradient image;
[0053] Step 2. Input the normalized processed image into the constructed fine-scale bone-suppressed convolutional neural network model in the intensity domain to obtain a fine-scale bone image;
[0054] Downsample the normalized image to the original size by 2 -s times to obtain a downsampled image, and then input the downsampled image into the coarse-scale bone-suppressed ...
Embodiment 2
[0088] A bone suppression method for lateral thoracic radiographs based on a deep convolutional neural network, specifically as follows:
[0089] Step 1. For the input original lateral chest image I 0 , using a Gaussian image filter with a large filter kernel to image I 0 Perform smoothing and filtering to get the base map I b , from image I 0 Subtract Basemap I b , to get the detail map I d . In order to make the input of the bone suppression model consistent, the details of Figure I d The gray value of the normalized processing, so that I d The mean value of the gray level in is 0 and the variance is 1. Detail map I after grayscale normalization d Input for the fine-scale bone suppression model in the intensity domain.
[0090] Step 2. Use the fine-scale bone-suppressed convolutional neural network model in the intensity domain, and use the normalized lateral chest image obtained in step 1 as input to predict the bone image of the lateral chest image I, and multiply...
PUM
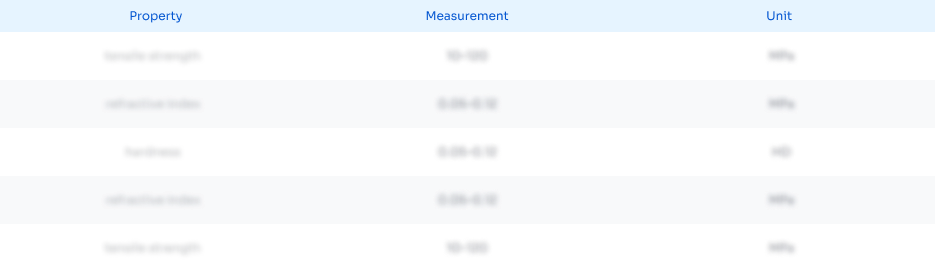
Abstract
Description
Claims
Application Information
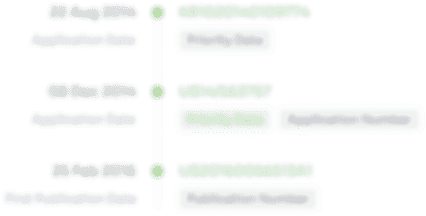
- R&D Engineer
- R&D Manager
- IP Professional
- Industry Leading Data Capabilities
- Powerful AI technology
- Patent DNA Extraction
Browse by: Latest US Patents, China's latest patents, Technical Efficacy Thesaurus, Application Domain, Technology Topic, Popular Technical Reports.
© 2024 PatSnap. All rights reserved.Legal|Privacy policy|Modern Slavery Act Transparency Statement|Sitemap|About US| Contact US: help@patsnap.com