Rolling bearing fault diagnosis method
A rolling bearing and fault diagnosis technology, which is applied in the testing of machines/structural components, testing of mechanical components, instruments, etc., can solve problems such as influence and achieve the effect of improving accuracy
- Summary
- Abstract
- Description
- Claims
- Application Information
AI Technical Summary
Problems solved by technology
Method used
Image
Examples
Embodiment 1
[0037] Embodiment 1: as figure 1 As shown, a rolling bearing fault diagnosis method first uses VMD to decompose the vibration signal of the rolling bearing into a series of modal components, calculate the sample entropy of each modal component and input it as a feature vector. Then, the cuckoo search algorithm is used to optimize the weight and threshold of the extreme learning machine, and the CS-ELM model is established. Finally, the sample entropy eigenvalue is input into the model to classify and identify the fault types of bearings under different working conditions.
[0038] The specific steps are:
[0039] 1) Collect the vibration signals of normal bearings, inner ring fault bearings, outer ring fault bearings, and rolling element faults of rolling bearings under a certain load state;
[0040] 2) Decompose the bearing fault vibration signal f(t) under different working conditions into k eigenmodes u k , while satisfying that the sum of the estimated bandwidths of eac...
Embodiment 2
[0063] Embodiment 2: In this embodiment, the method as shown in Embodiment 1 is adopted to carry out the fault diagnosis of the bearing, and the specific implementation steps are as follows:
[0064] Now install the acceleration sensor on the drive end of the rolling bearing. Among them, the bearing damage diameter is 0.1778mm, the set speed is 1797r / min, and the sampling frequency is 12kHz, and the different vibration signals of the rolling bearing under inner ring fault, outer ring fault, rolling element fault and normal state are measured respectively. Such as image 3 The waveform diagram shown.
[0065] Take 200 sets of data for each bearing state signal, 170 sets of data randomly selected from each state sample data, that is, as a training sample, the remaining four states of normal, inner ring fault, outer ring fault, and rolling element fault Each set of data of the bearing vibration signal is used as a test sample.
[0066] According to the principle of avoiding mo...
PUM
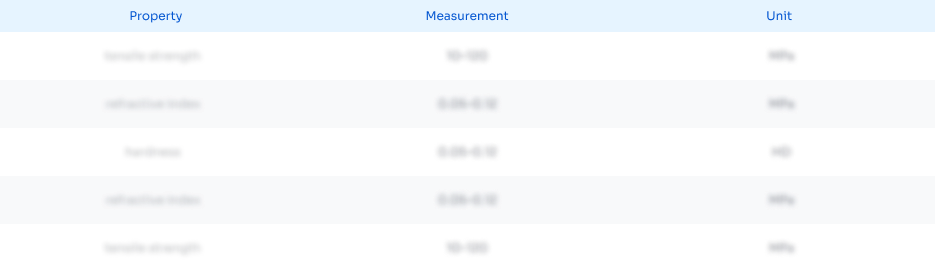
Abstract
Description
Claims
Application Information
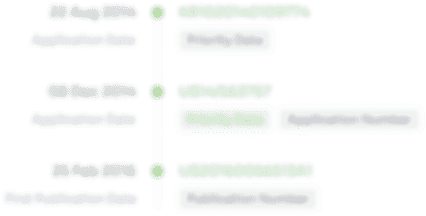
- R&D Engineer
- R&D Manager
- IP Professional
- Industry Leading Data Capabilities
- Powerful AI technology
- Patent DNA Extraction
Browse by: Latest US Patents, China's latest patents, Technical Efficacy Thesaurus, Application Domain, Technology Topic, Popular Technical Reports.
© 2024 PatSnap. All rights reserved.Legal|Privacy policy|Modern Slavery Act Transparency Statement|Sitemap|About US| Contact US: help@patsnap.com