Brain tumor segmentation method of deep residual network based on U-Net architecture.
A brain tumor and network technology, applied in the field of medical image segmentation and deep learning, can solve the problem of not fully considering the important role of residual learning, and achieve the effect of preventing network performance degradation, advanced performance, and improving network performance.
- Summary
- Abstract
- Description
- Claims
- Application Information
AI Technical Summary
Problems solved by technology
Method used
Image
Examples
Embodiment Construction
[0055] In order to make the objectives, technical solutions and advantages of the present invention more clearly understood, the present invention will be further described in detail below according to the accompanying drawings and examples.
[0056] The brain tumor segmentation process proposed by the present invention is a learning algorithm based on a convolutional neural network. The framework of the method consists of the following three main steps: 3D MRI data preprocessing, neural network training, and application of the trained algorithmic model to predict the structure of brain tumors.
[0057] A. Preprocessing
[0058] Before segmentation, it is usually necessary to preprocess the segmented brain images to eliminate the influence of irrelevant information, thereby improving the reliability of image segmentation results. In fact, even though MRIs from the same patient are all collected on the same scanner, MRIs can vary at different times or under pathological condit...
PUM
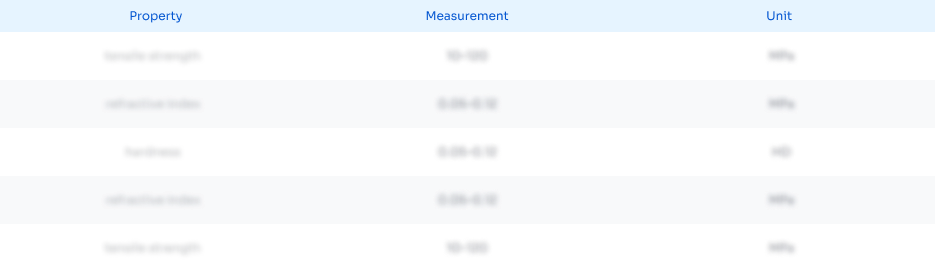
Abstract
Description
Claims
Application Information
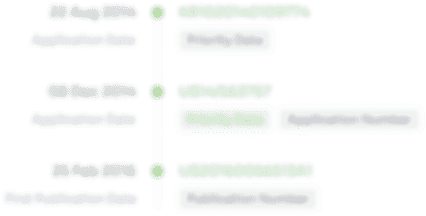
- R&D Engineer
- R&D Manager
- IP Professional
- Industry Leading Data Capabilities
- Powerful AI technology
- Patent DNA Extraction
Browse by: Latest US Patents, China's latest patents, Technical Efficacy Thesaurus, Application Domain, Technology Topic, Popular Technical Reports.
© 2024 PatSnap. All rights reserved.Legal|Privacy policy|Modern Slavery Act Transparency Statement|Sitemap|About US| Contact US: help@patsnap.com