Semi-supervised mechanical fault diagnosis method based on adaptive migration neural network
A neural network, mechanical failure technology, applied in neural learning methods, biological neural network models, neural architectures, etc., can solve problems such as not easy to implement
- Summary
- Abstract
- Description
- Claims
- Application Information
AI Technical Summary
Problems solved by technology
Method used
Image
Examples
Embodiment 1
[0066] This embodiment discloses a semi-supervised mechanical fault diagnosis method based on an adaptive migration neural network. The method uses a deep neural network G and an independent classifier C to learn on historical fault data and transfer the learned knowledge to the target task, thereby improving the diagnostic performance. like figure 1 As shown, the steps are as follows:
[0067] S1. Data acquisition and calibration: According to the actual mechanical equipment fault diagnosis task, use the sensor to obtain the source domain fault data of the corresponding mechanical equipment, so as to obtain multiple source domain fault data sets{X s , Y s}, where the source domain fault data set consists of the source domain fault training sample X obtained by intercepting the source domain fault data s and its corresponding label Y s Composition, the label is the fault type corresponding to the sample. Each fault data is intercepted based on the fault type and the princ...
Embodiment 2
[0114] This embodiment discloses a semi-supervised mechanical fault diagnosis device based on an adaptive migration neural network, such as Figure 5 shown, including:
[0115] The data acquisition and calibration module is used to obtain the source domain fault data of the corresponding mechanical equipment by using the sensor according to the actual mechanical equipment fault diagnosis task, so as to obtain multiple source domain fault data sets{X s , Y s}, where the source domain fault data set consists of the source domain fault training sample X obtained by intercepting the source domain fault data s and its corresponding label Y s Composition, the label is the fault type corresponding to the sample; according to different mechanical equipment, or the target domain fault data obtained under different working conditions or positions of the same mechanical equipment, construct the target domain fault data X without labels t The target domain fault data set is formed, and...
Embodiment 3
[0124] This embodiment discloses a storage medium, which stores a program. When the program is executed by a processor, the semi-supervised mechanical fault diagnosis method based on the adaptive migration neural network described in Embodiment 1 is implemented, specifically as follows:
[0125] S1. Data acquisition and calibration: According to the actual mechanical equipment fault diagnosis task, use the sensor to obtain the source domain fault data of the corresponding mechanical equipment, so as to obtain multiple source domain fault data sets{X s , Y s}, where the source domain fault data set consists of the source domain fault training sample X obtained by intercepting the source domain fault data s and its corresponding label Y s Composition, the label is the fault type corresponding to the sample; according to different mechanical equipment, or the target domain fault data obtained under different working conditions or positions of the same mechanical equipment, const...
PUM
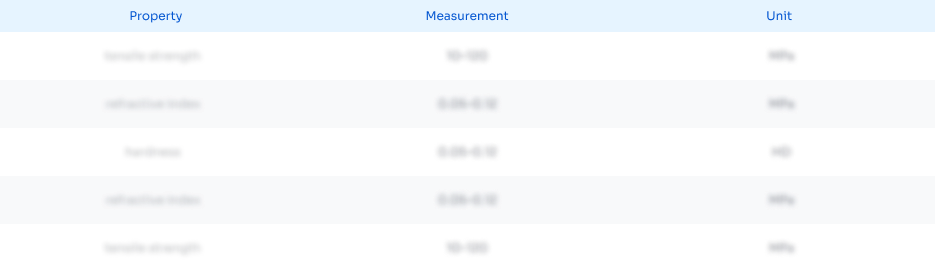
Abstract
Description
Claims
Application Information
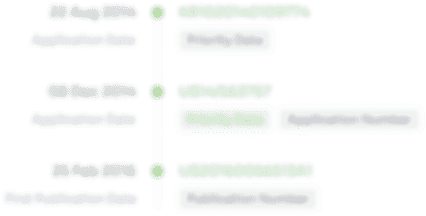
- R&D Engineer
- R&D Manager
- IP Professional
- Industry Leading Data Capabilities
- Powerful AI technology
- Patent DNA Extraction
Browse by: Latest US Patents, China's latest patents, Technical Efficacy Thesaurus, Application Domain, Technology Topic, Popular Technical Reports.
© 2024 PatSnap. All rights reserved.Legal|Privacy policy|Modern Slavery Act Transparency Statement|Sitemap|About US| Contact US: help@patsnap.com