Similarity-weight-semi-supervised-dictionary-learning-based human behavior identification method
A dictionary learning and similarity technology, applied in the field of pattern recognition, can solve the problems of difficulty in obtaining labeled samples, insufficient use of samples, and failure to consider the information of unlabeled samples.
- Summary
- Abstract
- Description
- Claims
- Application Information
AI Technical Summary
Problems solved by technology
Method used
Image
Examples
Embodiment Construction
[0047] refer to figure 1 , the present invention mainly includes three parts: dictionary learning, video representation, and video classification. The following are the implementation steps of these three parts:
[0048] 1. Dictionary learning
[0049] Step 1: Divide all video samples into training samples and test samples.
[0050] 1a) Input all video samples of the human behavior recognition dataset and their real labels i, select n video samples as training samples according to the method suggested by the author of the dataset, and the remaining h-n video samples in the dataset as test samples, where, i∈{1,2,...,c}, i represents the category label of the video sample, c represents the total number of category labels of the video sample, h represents the number of all video samples;
[0051] 1b) According to the real label i of the training samples in the data set, select w video samples from the video samples with the real label i as samples with known real labels, that ...
PUM
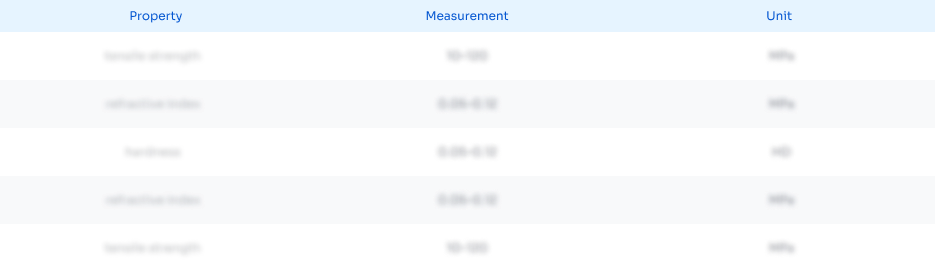
Abstract
Description
Claims
Application Information
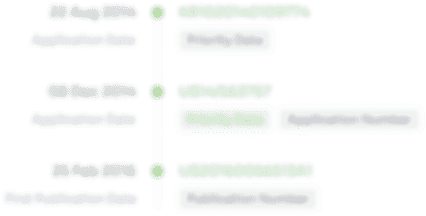
- R&D Engineer
- R&D Manager
- IP Professional
- Industry Leading Data Capabilities
- Powerful AI technology
- Patent DNA Extraction
Browse by: Latest US Patents, China's latest patents, Technical Efficacy Thesaurus, Application Domain, Technology Topic, Popular Technical Reports.
© 2024 PatSnap. All rights reserved.Legal|Privacy policy|Modern Slavery Act Transparency Statement|Sitemap|About US| Contact US: help@patsnap.com