A supervised fast discrete multimodal hash retrieval method and system
A multi-modal and supervised technology, applied in the field of cross-modal retrieval, can solve the problems of time-consuming and high computational complexity, and achieve the effect of enhancing discriminant, high learning efficiency, and avoiding computational complexity.
- Summary
- Abstract
- Description
- Claims
- Application Information
AI Technical Summary
Problems solved by technology
Method used
Image
Examples
Embodiment 1
[0054] This embodiment discloses a supervised fast discrete multimodal hash retrieval method, comprising the following steps:
[0055] Step 1: Obtain the multimodal training data set O train , where each sample contains pairs of multimodal data features, such as image and text;
[0056] Step 2: Using the joint multimodal feature map, the multimodal training dataset O train Projecting to a joint multimodal intermediate representation;
[0057] Described step 2 specifically comprises:
[0058] First, the multimodal training data set O train The data features of each modality in are transformed into a nonlinear embedding φ m (x (m) ):
[0059]
[0060] Among them, {x (m)} m=1,...,M is the training data set of the mth mode, and there are M modes in total, is the anchor point set (we randomly select a part of the samples in the training samples of the corresponding modality to form the anchor point set), N is the total number of training samples of the modality, P is t...
Embodiment 2
[0117] The purpose of this embodiment is to provide a computer system.
[0118] A computer system, comprising a memory, a processor, and a computer program stored in the memory and operable on the processor, when the processor executes the program, it realizes:
[0119] Receive a multimodal training dataset, where each sample contains pairs of multimodal data features;
[0120] Using the joint multimodal feature map, project the multimodal training dataset into a joint multimodal intermediate representation;
[0121] For the joint multimodal intermediate representation of the multimodal training data set, construct a supervised fast discrete multimodal hash objective function; solve the objective function to obtain a hash function;
[0122] Receive the multimodal retrieval data set and the multimodal test data set, project the samples in it into a joint multimodal intermediate representation, and then project to the Hamming space according to the hash function to obtain the h...
Embodiment 3
[0124] The purpose of this embodiment is to provide a computer-readable storage medium.
[0125] A computer-readable storage medium, on which a computer program is stored, and when the program is executed by a processor, the following steps are performed:
[0126] Receive a multimodal training dataset, where each sample contains pairs of multimodal data features;
[0127] Using the joint multimodal feature map, project the multimodal training dataset into a joint multimodal intermediate representation;
[0128] For the joint multimodal intermediate representation of the multimodal training data set, construct a supervised fast discrete multimodal hash objective function; solve the objective function to obtain a hash function;
[0129] Receive the multimodal retrieval data set and the multimodal test data set, project the samples in it into a joint multimodal intermediate representation, and then project to the Hamming space according to the hash function to obtain the hash co...
PUM
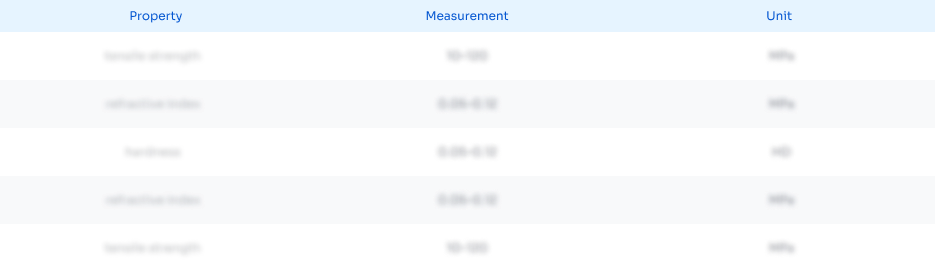
Abstract
Description
Claims
Application Information
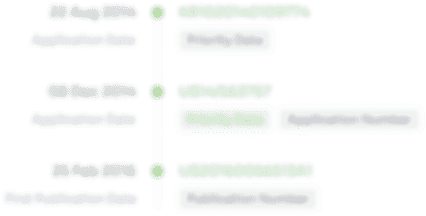
- R&D Engineer
- R&D Manager
- IP Professional
- Industry Leading Data Capabilities
- Powerful AI technology
- Patent DNA Extraction
Browse by: Latest US Patents, China's latest patents, Technical Efficacy Thesaurus, Application Domain, Technology Topic, Popular Technical Reports.
© 2024 PatSnap. All rights reserved.Legal|Privacy policy|Modern Slavery Act Transparency Statement|Sitemap|About US| Contact US: help@patsnap.com